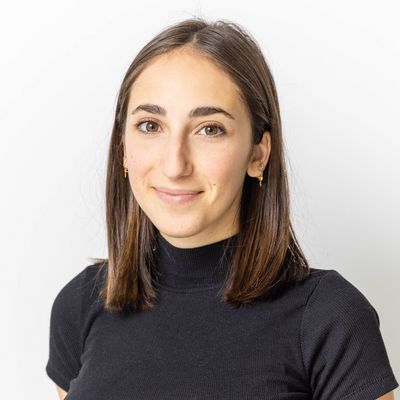
Ekin Celikkan
GFZ - KIT
Contact
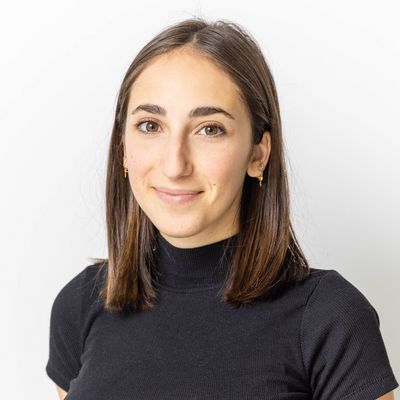
Supervisors:
Weeds are significant contributors (about 12% of global crop production) to crop yield and quality decline. Farmers use different approaches such as chemical or biological herbicides to eliminate weeds. However, excess use of herbicides leads to the pollution of soils, water, and air, putting the above and below ground wildlife biodiversity at risk. Alternative weed mitigation strategies must be designed and promoted. The site-specific weed management (SSWM) approach has been proposed consisting of varying weed management strategies within a crop field to suit the weed population's variation in density, location, and composition. The first step in implementing a SSWM strategy is accurate and timely detection and mapping of weeds. The high temporal, spatial, and spectral remote sensing information is required to capture detailed within-field variability, which can only be met by using the Low Altitude Remote Sensing platform and lightweight hyperspectral imaging sensors that can be deployed locally and at varying conditions.
This research aims to use hyperspectral multi-temporal Low altitude remote-based time-series imagery combined with field data and advanced machine learning (ML) techniques to detect and discriminate weeds in croplands. To achieve this aim, the project will i) monitor the weed population during different growth stages and preprocess of hyperspectral data and field data for better detecting weeds, ii) test a combination of ML and image processing algorithms for detecting weeds across a range of field and growing stage conditions, iii) apply the framework for multi-temporal analysis to track weed distribution and conditions for theuptake by precision farming techniques in our study regions.
Full-length publications
- E. Celikkan, M. Saberioon, M. Herold and N. Klein (2023). Semantic Segmentation of Crops and Weeds with Probabilistic Modeling and Uncertainty Quantification. Proceedings of the IEEE/CVF International Conference on Computer Vision (ICCV) Workshops, pp. 582-592.
- E. Celikkan, T. Kunzmann, Y. Yeskaliyev, S. Itzerott, N. Klein, and M. Herold (2025). WeedsGalore: A Multispectral and Multitemporal UAV-Based Dataset for Crop and Weed Segmentation in Agricultural Maize Fields. Proceedings of the Winter Conference on Applications of Computer Vision (WACV), pp. 4767-4777.
Conference presentations
-
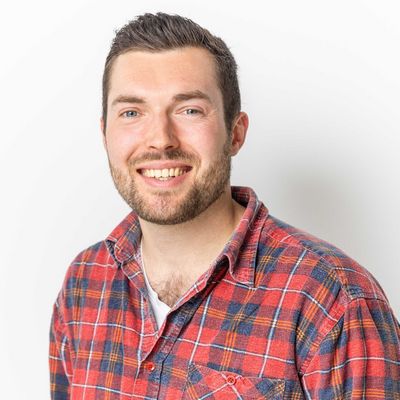
Daniel Collin
GFZ - TU Berlin
Contact
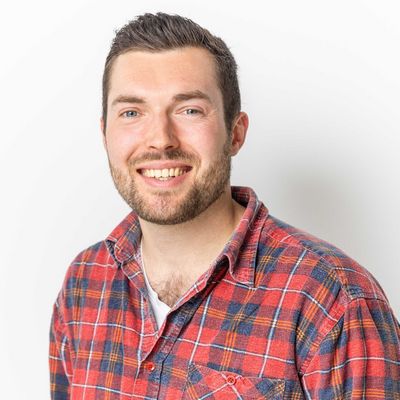
Supervisors:
Space weather is a term used to describe hazardous events in the near-Earth space environment that can have adverse effects. Power grids, telecommunication infrastructure and space assets show significant vulnerability to space weather events originating from the Sun. While these effects are largely invisible to the naked eye, in the 21st century operations in space and on the ground significantly depend on the accurate knowledge and forecast of the conditions in the space environment.
Using ground observations of the Sun and physics-based models of the Solar System, it is possible to quantify space weather hazards (e.g., solar wind speed and density) and risks on Earth’s technology. The output of this method provides an estimate of the desired variables. These methods, however do not utilize the vast amount of observations that are available from space, and they are computationally demanding and dominantly physics-based, making them difficult to run in real-time and use all available measurements.
We propose to leverage the growing number of highly detailed multi-spectral images of the Sun to improve predictions of the solar wind streams arriving at the Earth. We propose to exploit the capabilities of modern computer vision and machine learning (ML) techniques to register solar images, analyze them and assimilate them in an empirical (data-driven) model. Through our approach we will develop a novel, data-driven framework directly connecting solar disturbances to its consequences for space weather.
Full-length publications
- D. Collin, Y. Shprits, S.J. Hofmeister, S. Bianco, and G. Gallego (2025). Forecasting High-Speed Solar Wind Streams from Solar Images. Space Weather. https://doi.org/10.1029/2024SW004125
Conference presentations
- D. Collin, S. Bianco, G. Gallego, and Y. Shprits. Forecasting solar wind speed from solar EUV images. (Oral and poster presentation), International Workshop on Machine Learning and Computer Vision in Heliophysics, Sofia, Bulgaria, 19-21 April 2023.
- D. Collin, S. Bianco, G. Gallego, and Y. Shprits. Forecasting solar wind speed by machine learning based on coronal hole characteristics. (Poster presentation), EGU General Assembly , Vienna, Austria, 24–28 April 2023. https://doi.org/10.5194/egusphere-egu23-6968
- D. Collin, S. Bianco, G. Gallego, and Y. Shprits. Forecasting solar wind speed from solar EUV images. (Oral presentation), IUGG General Assembly, Berlin, Germany, 11-20 July 2023. https://doi.org/10.57757/IUGG23-2070
- D. Collin, S. Bianco, G. Gallego, and Y. Shprits. Forecasting solar wind speed from coronal holes. (Oral presentation), AGU Fall Meeting, San Francisco, USA, 11–15 December 2023.
- D. Collin, Y. Shprits, S. Bianco, F. Inceoglu, S. Hofmeister, and G. Gallego. Forecasting solar wind speed from coronal holes and active regions. (Poster presentation), EGU General Assembly, Vienna, Austria, 14–19 April 2024. https://doi.org/10.5194/egusphere-egu24-18676
- D. Collin, Y. Shprits, S. Hofmeister, S. Bianco, and G. Gallego. Forecasting solar wind speed with solar images. (Oral and poster presentation), International Magnetosphere Coupling Workshop IV, Potsdam, Germany, 3–7 June 2024.
- D. Collin, Y. Shprits, S. Bianco, S. Hofmeister, and G. Gallego. Forecasting Solar Wind Speed from Solar EUV Images. (Poster presentation), Helmholtz AI Conference, Düsseldorf, Germany, 12-14 Jun 2024.
- D. Collin, Y. Shprits, S. Bianco, S. Hofmeister, and G. Gallego. Using Distributional Regression to Improve Solar Wind Speed Forecasting from Solar Images. (Oral presentation), European Space Weather Week, Coimbra, Portugal, 4-8 Nov 2024.
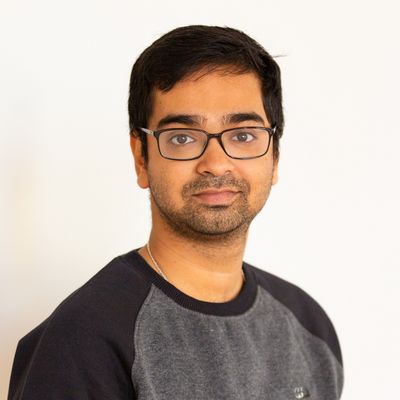
Binayak Ghosh
GFZ - Uni Tübingen
Contact
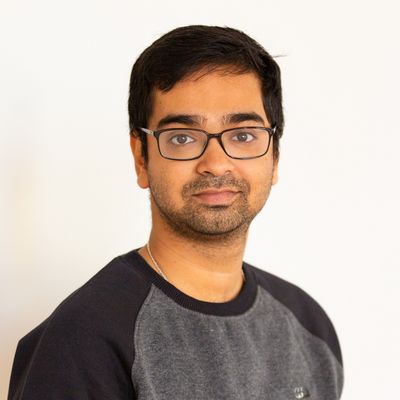
Supervisors:
Setareh Maghsudi (Uni Tübingen)
Enabled by recent technological advances, the field of radar remote sensing has entered the era of explosively-growing wide-swath Synthetic Aperture Radar (SAR) missions with short revisit times (1-6 days), such as Sentinel-1 and the planned Tandem-L and NISAR missions, providing an unprecedented wealth of topography and surface change time-series using interferometric SAR (InSAR) technique. Such data volume can be characterized with (i) huge volume and large variety; (ii) complexity and high dimension; (iii) partial unreliability; and (iv) correlation or similarity. Thus, for the retrieval of geophysical signal from InSAR time-series, the data should be classified, clustered and cleaned, performed by data analytics, to reliably detect anomaly changes and improve susceptibility in the areas affected by deformation due to natural and manmade hazards. In the state of- the-art literature for exploiting InSAR time-series data, the separation of geophysical signals from noise artifacts such as atmosphere and decorrelation can be divided into two main parts: (i) data processing for efficient estimation of the phase considering long stack of the data; and (ii) data analysis and decision.
In brief, the main goal of this project is to develop a generic framework for real-time InSAR data analytics using the theory and methods from online machine learning and sequential decision making, and to design efficient algorithmic solutions for the retrieval of geophysical signals from SAR measurement. In particular, the concentration is on SAR data from Sentinel-1 satellite. On the online learning and classification side, the methodology is concentrated on online machine learning algorithms. Specific attention is given to submodular optimization. On the online decision making side, the basic method is sequential optimization with limited feedback, especially multi-armed bandit.
Full-length publications
-
B. Ghosh, M. Motagh, M. Haghshenas Haghighi, M. Stefanova Vassileva, T. Walter, and S. Maghsudi (2021). Automatic detection of volcanic unrest using blind source separation with a minimum spanning tree based stability analysis. IEEE Journal of Selected Topics in Applied Earth Observations and Remote Sensing. https://doi.org/10.1109/JSTARS.2021.3097895
- B. Ghosh, M. Haghshenas Haghighi, M. Motagh, and S. Maghsudi (2021). Using Generative Adversarial Networks for extraction of InSAR signals from large-scale Sentinel-1 Interferograms by improving tropospheric noise correction. ISPRS Ann. Photogramm. Remote Sens. Spatial Inf. Sci., V-3-2021, 57–64. https://doi.org/10.5194/isprs-annals-V-3-2021-57-2021
- B. Ghosh, S. Garg, and M. Motagh (2022). Automatic flood detections from Sentinel-1 data using deep learning architectures. ISPRS Ann. Photogramm. Remote Sens. Spatial Inf. Sci., V-3-2022, 201–208. https://doi.org/10.5194/isprs-annals-V-3-2022-201-2022.
- B. Ghosh, S. Garg, M. Motagh, and S. Martinis (2024). Automatic Flood Detection from Sentinel-1 Data Using a Nested UNet Model and a NASA Benchmark Dataset. PFG, 92, 1–18. doi.org/10.1007/s41064-024-00275-1
Conference presentations
- B. Ghosh, M. Motagh, S. Maghsudi, and M.H. Haghighi. Reduction of tropospheric noise delay from large-scale interferograms using Generative Adversarial Networks. (Oral presentation), 40th Annual Scientific and Technical Conference of the DGPF, Stuttgart, Germany, 4 - 6 March 2020.
-
B. Ghosh, M. Motagh, S. Maghsudi, and M.H. Haghighi. Automatic flood monitoring based on SAR intensity and interferometric coherence using machine learning. (Oral presentation), EGU General Assembly, Online, 4-8 May, 2020.
-
B. Ghosh, M. Motagh, M.H. Haghighi, and T. Walter. Using minimal spanning tree based ICA optimization for volcanic unrest determination. (Oral presentation), EGU General Assembly, Online, 19-30 April, 2021.
-
B. Ghosh, M.H. Haghighi, M. Motagh, and S. Maghsudi. Using generative adversarial networks for extraction of InSAR signals from large-scale Sentinel-1 interferograms by improving tropospheric noise correction. (Oral presentation), ISPRS Congress, 4-10 July 2021.
- B. Ghosh, M. Motagh, S. Garg, M. Sips, and D. Eggert. Deep learning, remote sensing and visual analytics to support automatic flood detection. (Oral presentation), EGU General Assembly, Vienna, Austria & Online, 23-27 May 2022.
- B. Ghosh, S. Garg, M. Motagh. Automatic flood detection from Sentinel-1 data using Deep learning architectures. (Oral presentation), ISPRS Congress, Nice, France, 6-11 June 2022.
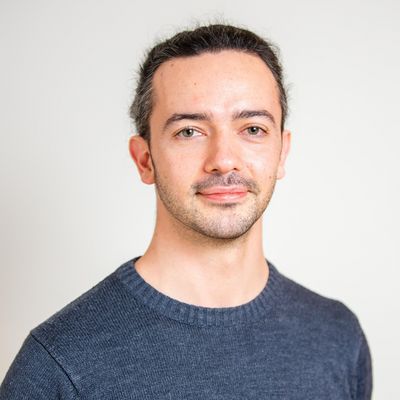
Paolo Graniero
FU Berlin - HZB
Contact
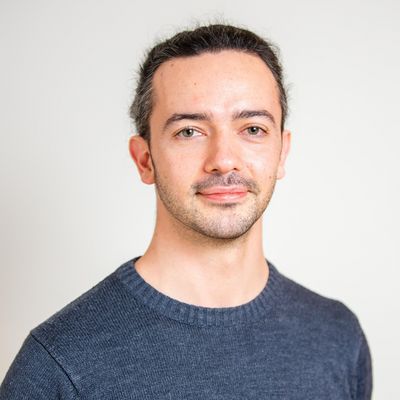
Supervisors:
The number of photovoltaic (PV) systems installed worldwide is steadily increasing, along with their share in the energy produced in the power grid. Getting the maximum benefit from these PV systems involves two primary considerations: maximizing power generation over the entire lifetime of the PV modules and optimally integrating them into the power grid.
Getting the maximum output from a given PV installation requires continuous monitoring to detect underperformance and failures as early as possible. However, this type of monitoring is seldom implemented, particularly outside of utility-scale photovoltaic plants or research-focused installations. These facilities are equipped with all the instruments required for accurate monitoring, in contrast to residential and commercial installations.
The capabilities of modern data analytic methods can help raise the proportion of PV installations that can be monitored. In addition, data-driven modeling can make monitoring a PV system simpler: data-driven models do not require detailed information about the system, and they can also combine data from sources that are available outside of plants and research centers, but which standard, physics-based modeling approaches can't take advantage of.
One thing that could hinder the integration of PV systems into the power grid is the stochastic nature of solar energy. This stochasticity could lead to adverse effects such as over- and under-production and challenge the stability of power grids. This means that in addition to monitoring, the integration will also require the most accurate possible forecasting of expected power to control the grid optimally and avoid unnecessary stresses on it. Data-driven methodologies can help make the implementation of such best practices more accessible and more widely available, benefiting both PV system owners and power grid managers.
In this project, we investigate data-driven monitoring and forecasting systems for photovoltaic installations. As an application, we study the potential and benefits of PV power monitoring and forecasting for load management in support of electric mobility integration in the public transport system. We focus specifically on electric buses and the load generated from charging their batteries. Suppose the charging tasks are performed in an uncontrolled way. In that case, the large electric loads of the batteries might cause instability of the power grid and reduce the environmental benefits of such electric vehicles. Contrary to this, load management coordinates the charging processes to benefit as much as possible from renewable energy sources while preserving the power grid stability.
The coordination of energy generation from PV systems and energy consumption by electric vehicles is a key to a green transition in the transport sector.
Full-length publications
- P. Graniero, M. Khenkin, H. Köbler, N.T. Putri Hartono, R. Schlatmann, A. Abate, E. Unger, T.J. Jacobsson and C. Ulbrich (2023). The challenge of studying perovskite solar cells’ stability with machine learning. Front. Energy Res., Sec. Solar Energy, 11. https://doi.org/10.3389/fenrg.2023.1118654
- N.T.P. Hartono, H. Köbler, P. Graniero, et al. (2023). Stability follows efficiency based on the analysis of a large perovskite solar cells ageing dataset. Nat Commun, 14, 4869. https://doi.org/10.1038/s41467-023-40585-3
Conference presentations
- P. Graniero, A. Louwen, R. Schlatmann, and C. Ulbrich. Comparison of different data sources for Machine Learning algorithms in photovoltaic output power estimation. (Poster presentation), 37th EU PVSEC, Online, 7-11 September 2020.
- P. Graniero, D. Rößler, C. Ulbrich, and N. Kliewer. Potentials and challenges for integration of electric bus fleets and PV-systems. (Oral presentation), 31st European Conference on Operational Research (EURO 2021), Athens, Greece / Online, 11-14 July 2021.
- P. Graniero. Data driven mitigation measures in advanced PV plant monitoring. (Oral presentation), Intersolar Conference, Munich, 6-7 October 2021.
- P. Graniero. Comparison of Unsupervised Algorithms for PV Fault Detection, and Data Sources for Power Nowcasting. (Oral presentation), Cost Action Pearl PV’s Conference- Enabling the Terawatt Transition, Enschede, The Netherlands, 14-16 March 2022.
- P. Graniero, G.A.F. Basulto, R. Schlatmann, R. Klenk, and C. Ulbrich. Online Implementation of a Multiple Linear Regression Model for CIGS Photovoltaic Module Performance. (Poster presentation), WCPEC-8, Milan, Italy, 26-30 September 2022.
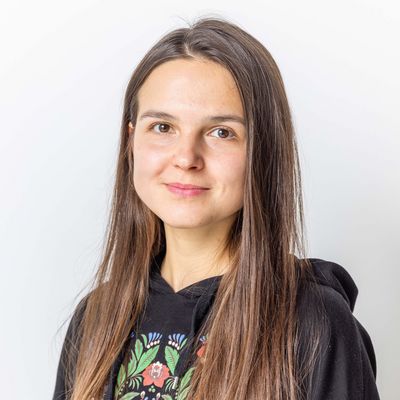
Viktoriia Huryn
MDC - Charité
Contact
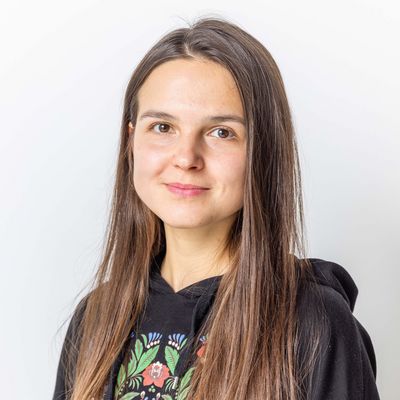
Supervisors:
Markus Schuelke-Gerstenfeld (Charité)
Single-cell genomics can obtain molecular data for tens of thousands of cells simultaneously. A typical experiment is carried out on a complex sample that contains different cell types, and can measure different cellular properties, such as the number of messenger RNA molecules per cell. Typical tasks include identifying distinct cell types (e.g. via unsupervised embeddings, [1]) or inferring a pseudo-temporal ordering of cells along developmental stages. A particular opportunity arises from single-cell genome accessibility data, which provides information about which of several million gene switches, so called regulatory regions, are accessible/on or inaccessible/off [2]. These data can be analyzed at multiple resolutions: At the level of whole regions, to identify where active switch regions are and to infer which genes they may regulate, or at the level of short DNA sequence patterns within the regions, which are recognized by proteins to specifically activate the switches in e.g. different cell-types. Models to utilize the power of single-cell genomics data, and accessibility in particular, are still in their infancy. The main challenge is that the higher number of cells (i.e. samples) is accompanied by high dropout: the readout covers only a few percent of all variables, and the resulting discrete count data is sparse. Additionally, ground truth experimental data only exists for a handful of scenarios, making it hard to develop practically useful methods that work beyond simulated data.
The project will utilize data from the Schuelke lab to develop deep neural network approaches in the Ohler lab that enable flexible multi-resolution analyses: the goal is to devise models that are able to infer both active regulatory regions and the functional sequence patterns in them, while (a) leveraging data from smaller or larger cell neighborhoods as needed; (b) accounting for confounders such as variable dropout and cell type mixtures; and (c) utilizing auxiliary data from other single-cell experiments.
Full-length publications
-
Conference presentations
- V. Huryn, R. Monti, A.A. Rakowski, and V. Döring. Disentanglement learning for functional genomics data. (Poster presentation), Kipoi Summit. New Horizons in Computational Regulatory Genomics. Zugspitze, Germany, 25-26 September 2023.
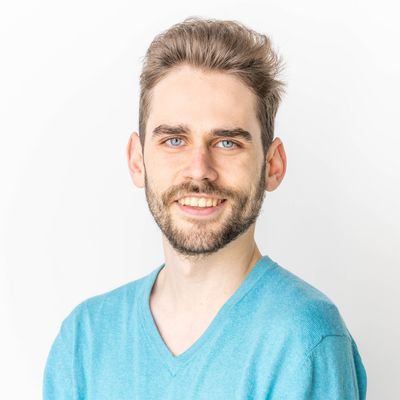
Daniel León Periñán
MDC - TU Berlin - Charité
Contact
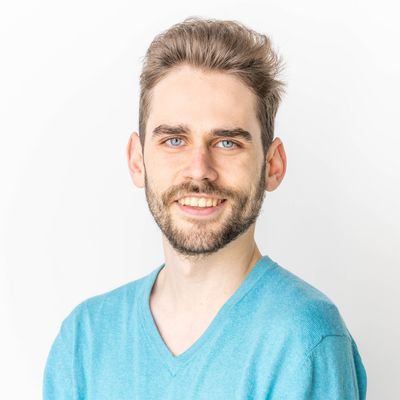
Supervisors:
Despite enormous progress in the understanding, early diagnosis and treatment, solid tumors still account for a quarter of deaths. Solid tumors arise from somatic cells through the accumulation of molecular alterations that eventually lead to their uncontrolled proliferation, invasion of healthy tissues and ultimately, distant metastases. In current clinical practice, the best treatment is chosen by assessing clinical presentation, histological tumor type and molecular characteristics, such as oncogenic mutations and, in some cases, gene expression profiles. The evaluation of histomorphological tumor properties in combination with molecular profiles guides risk-adjusted, personalized therapies that aim to optimize outcome for individual patients. However, diagnostic molecular profiling currently mostly relies on techniques performed on bulk tissue without providing any spatial information about the observed molecular tumor properties. While this can already make the interpretation of mutational profiles challenging, spatially resolved profiling becomes indispensable for the molecular analysis of the tumor microenvironment composed of multiple different cell types whose complex interactions influence therapy response. In this context, single-cell sequencing techniques may offer a unique opportunity to offer both high spatial and molecular resolution in the context of complex tumor histology.
Recently, the fruitful interdisciplinary collaboration between clinical, technological, and computational researchers has cultivated rapid progress in the field of digital pathology. AI-based models provide support to diagnostic pathology, for instance by automating tumor identification and tissue classification and cell detection from tumor histology images. Current computational models, however, are limited in their ability to predict tumor molecular characteristics due to the scarcity of paired imaging and molecular training data. Development of spatial transcriptomics assays aim to fill this gap by enabling unbiased, transcriptome-wide profiling of mRNA expression in intact tissue sections. Thus, they represent an ideal source of such paired morphological and molecular data with unprecedented resolution for training the next generation of digital pathology algorithms.
The project aims to push forward the field of digital pathology by predicting gene expression in tissue space from histomorphology alone. To achieve that, we will train deep learning models on the high-resolution gene expression maps provided by spatial transcriptomics co-registered with the corresponding histomorphology images. Advances in explainable AI approaches will be leveraged to reveal which morphological features and areas are exploited by such models to predict gene expression. This would be vital to allow for a transparent decision process, crucial for medical applications, and would also deepen our understanding of tumor biology by correlating tissue and cellular composition/histomorphology of the tumor and its microenvironment with function. We anticipate such a computational approach to have a positive impact on clinical practices by facilitating the prediction of molecular properties from routine diagnostic H&E images and thus to complement or even partially replace molecular testing.
Full-length publications
- T.M. Pentimalli, S. Schallenberg, D. León-Periñán, ..., F. Klauschen, and N. Rajewsky (2025). Combining spatial transcriptomics and ECM imaging in 3D for mapping cellular interactions in the tumor microenvironment. Cell Systems. https://doi.org/10.1016/j.cels.2025.101261
- M. Schott, D. León-Periñán,.., T.M. Pentimalli, …, N. Karaiskos, and N. Rajewsky (2024). Open-ST: High-resolution spatial transcriptomics in 3D. Cell. https://doi.org/10.1016/j.cell.2024.05.055
Conference presentations
- D. León-Periñán, N. Karaiskos, M. Schott, E. Splendiani, E. Senel, and N. Rajewsky. Computational methods for high-resolution spatial transcriptomics. (Poster presentation), VIB Spatial Omics, Ghent, Belgium, 13-14 June 2024.
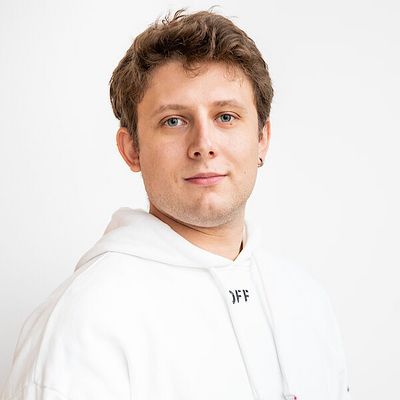
Oleksii Martynchuk
DLR - TU Berlin
Contact
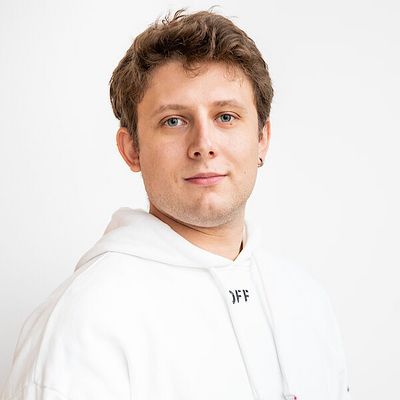
Supervisors:
Mars has kilometer‐thick polar ice caps, which represent a unique laboratory to study the dynamics of ice sheets and the possible effects of climate change on a planet other than Earth. Most of the north polar cap margins are characterized by steep scarps, where avalanches and ice‐block falls are frequently observed. These cause a measurable scarp retreat, depending on season and the associated solar heating cycle. Rock falls also represent useful sources for seismic experiment and the exploration of the subsurface of the planet. The High‐Resolution Imaging Science Experiment (HiRISE) on-board the Mars Reconnaissance Orbiter (MRO) has been monitoring the planet for more than a decade, returning images at resolutions up to 25 cm, many of which in stereo. However, the identification of such small “mass wasting” effects, such as block and rock falls, is far from trivial considering the vast number of images in their differing illumination and viewing geometry. The image analysis requires new approaches of automated detection involving machine learning, which go beyond the traditional classification and regression schemes. In this project we apply modern data science techniques, such as deep convolutional networks to identify and measure rock sizes and volumes. The techniques are to be trained on established areas and ultimately be applied to new sets of images to investigate the recent climate evolution and support the seismic exploration of the planet.
Full-length publications
-
Conference presentations
-
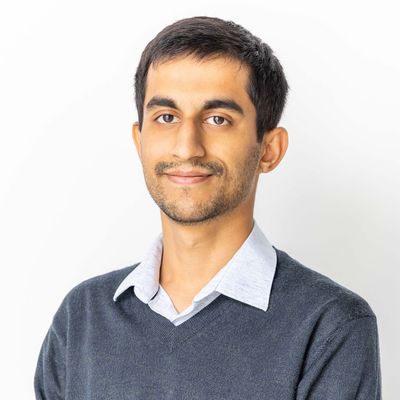
Abhay Mehta
DESY - HU Berlin
Contact
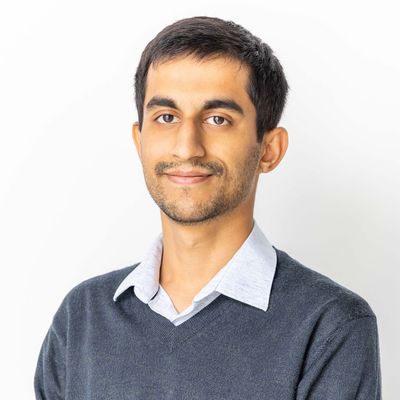
Supervisors:
The sensitivity of ground-based gamma-ray telescopes is ultimately limited by their ability to reconstruct the properties of gamma-rays from the particle shower produced when they interact with the atmosphere and reject the much more numerous background of showers from charged cosmic rays.
At this time, array sensitivity can be considered as “software limited” and as such has continuously improved in the past 20 years by exploiting advanced image reconstruction and classification algorithms [1] and multivariate classification techniques such as boosted decision trees [2] and neural networks. It is clear that using modern machine learning techniques can improve this performance further. Yet, telescope arrays typically make observations under a huge range of context conditions. As such, it is of utmost importance to integrate contextual data on the operation of the telescope as well as on atmospheric conditions into any data analysis pipeline. This is challenging not only because of the heterogeneity of contextual data, but also from a computational point of view.
This PhD project sets out to develop data processing pipelines that combine deep learning techniques for gamma-ray telescope data with diverse types of contextual data to dramatically improve the telescopes’ sensitivity. To this end, we will draw on initial results on employing state-of-the-art machine learning techniques to gamma-ray telescope data [3] as well as technical insights into integration of static datasets into stream processing pipelines [4].
Full-length publications
-
Conference presentations
- A. Mehta. Machine Learning for Imaging Atmospheric Cherenkov Telescope (IACT) Background Rejection. H.E.S.S. Collaboration Meeting, Bordeaux, France, 24-29 September 2023.
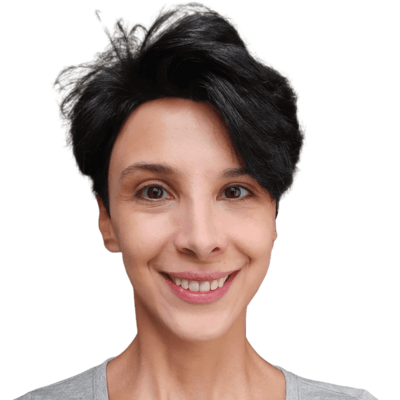
Lusinè Nazaretyan
Charité - MDC - HU Berlin
Contact
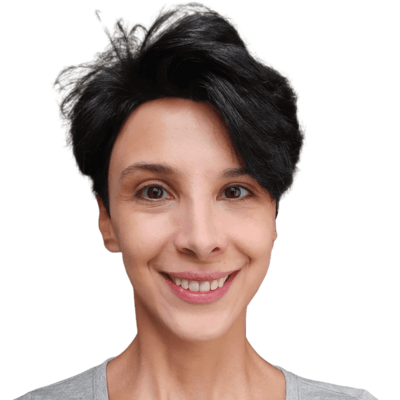
Supervisors:
More than 15 years after initial sequencing of the human genome, exome and whole genome sequencing are widely performed for research and clinical applications. Despite much progress, pinpointing the few phenotypically causal variants among the millions of variants in our genomes remains a major challenge. To illustrate that problem, the NCBI dbSNP and ClinVar databases report almost 700 million variants discovered in healthy and diseased humans, but only about 500,000 (<0.1%) are clinically or functionally characterized. For diagnostics, it is critical to identify the causal variants among thousands of variants with no physiological effect.
Many algorithms for predicting functional impact of variants were proposed in the past, but are largely limited to highly conserved positions in protein-coding sequences and do not interpret variants genome wide. Kircher et al. previously developed a computational method (Combined Annotation Dependent Depletion, CADD) that combines diverse annotations – from large-scale epigenetic experiments, comparisons of genomes across species, to gene model annotations. Using a linear model, CADD integrates available information in a unified framework and quantifies organismal deleteriousness on a whole-genome and variant-specific scale. While CADD has been successfully applied in thousands of disease studies, its best performance is still observed for the interpretation of variants in and around protein coding genes.
Reasons for that are manifold, ranging from the lack of domain-specific features (e.g. non-coding sequence species, regulatory sequences, 3D genome architecture) to shortcomings in the actual model (e.g. non-linearity, missing feature interactions, mislabeling of the training data). Here, we propose a joint effort, between a group that routinely analyses and interprets genetic data from individual patients, families or large research cohorts and a research group developing computational methods for variant prioritization, to significantly improve the current method and advance the automatic reporting of potentially clinically relevant variants from genetic data. For this purpose, our project has the following aims: (1) Establishing model training for unlabeled or mislabeled data, for example by semi-supervised and iterative learning approaches. (2) Systematic exploration of feature interactions and non-linearity, including but not limited to alternative learning approaches like boosting trees and neural networks, feature transformations, or automated selection of interactions terms. (3) Integration of large sets of correlated annotations (e.g. ENCODE, IHEC) through dimensionality reduction, orthogonalization approaches, parallelization and training via subsampling, or hierarchical integration of models. (4) Using additional and genome-wide available measures of sequence constraint like population variant density and sequence-dependent mutational load, to complement species conservation.
Full-length publications
- M. Schubach, L. Nazaretyan and M. Kircher (2023). The Regulatory Mendelian Mutation score for GRCh38. GigaScience, 12, giad024. https://doi.org/10.1093/gigascience/giad024
- M. Schubach, T. Maass, L. Nazaretyan, S. Röner, and Martin Kircher (2024). CADD v1.7: Using protein language models, regulatory CNNs and other nucleotide-Level scores to improve genome-wide variant predictions. Nucleic Acids Research, 52, D1, D1143-D1154. https://doi.org/10.1093/nar/gkad989
Conference presentations
- L. Nazaretyan, M. Schubach, and M. Kircher. The Regulatory Mendelian Mutation (ReMM) score for GRCh38. (Poster presentation), ISMB/ECCB 2021, Online, 25-30 July, 2021.
- L. Nazaretyan, M. Schubach, and M. Kircher. The Regulatory Mendelian Mutation (ReMM) score for GRCh38. (Poster presentation), ESHG 2021, Online, August 28–31, 2021.
- L. Nazaretyan, M. Kircher, and U. Leser. Benchmarking machine learning methods for identification of mislabeled data. (Poster presentation), ECCB 2022, Sitges, Spain, September 18-21, 2022.
- M. Schubach, T. Maass, L. Nazaretyan, S. Röner, and M. Kircher. CADD v1.7: Using protein language models, regulatory CNNs and other nucleotide-level scores to improve genome-wide variant predictions. (Poster presentation), Genome Informatics, Cold Spring Harbour, 6-9 December 2023.
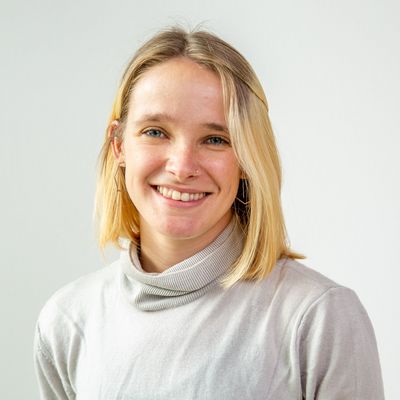
Elizabeth Robertson
DLR - TU Berlin
Contact
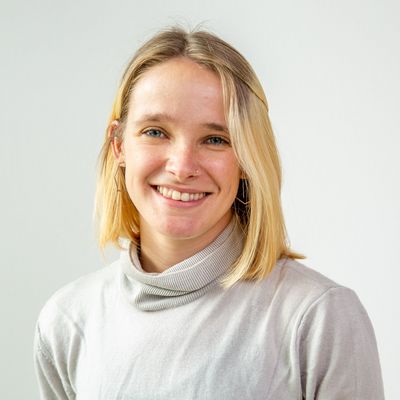
Supervisors:
Classical digital computer architectures are visibly approaching their technological and physical limits. Thus, there is a growing interest in developing post-digital computing approaches to overcome these limitations. Besides quantum computers, approaches that emulate neuromorphic processes represent a promising alternative because they mimic the massively parallel, energy-efficient computations carried out by the human brain. Such computations constitute the building blocks of the pattern recognition algorithms underpinning the success of machine learning (ML). Optically integrated systems promise 2–3 orders of magnitude higher energy efficiency compared to today's electronic approaches. Among others, post-digital computer concepts will enable numerous new applications for ML in places like data centers or security systems, as well as autonomous vehicles, drones and satellites – any area where massive amounts of computations need to be done but is limited by power and time. In this project we will realize ML with optical neural networks. That is, we want to use light to power machine learning, due to the potential advantages that an optical neural network (ONN) has over one that is emulated on conventional GPU chips. Moreover, we will investigate the potential of neuromorphic computing hardware for ML on low-power autonomous systems.
Full-length publications
- L. Jaurigue, E. Robertson, J. Wolters, and K. Lüdge (2021). Reservoir computing with delayed input for fast and easy optimisation. Entropy, 23, 1560. https://doi.org/10.3390/e23121560
- M. Yang, E. Robertson, L. Esguerra, K. Busch and J. Wolters (2023). Optical convolutional neural network with atomic nonlinearity. https://doi.org/10.48550/arXiv.2301.09994 [Preprint]
- L. Meßner, E. Robertson, L. Esguerra, K. Lüdge and J. Wolters (2023). Multiplexed random-access optical memory in warm cesium vapor. https://doi.org/10.48550/arXiv.2301.04885 [Preprint]
- E. Robertson, L. Esguerra, L. Meßner, G. Gallego, and J. Wolters (2024). Machine-learning optimal control pulses in an optical quantum memory experiment. Phys. Rev. Applied, 22, 024026. https://doi.org/10.1103/PhysRevApplied.22.024026
Conference presentations
- E. Robertson, L. Jaruingue, L. Messner, L. Esguerra, G. Gallego, K. Lüdge, and J. Wolters. A scheme for optical reservoir computers with atomic memory. (Poster presentation), Hot Vapor Workshop, Stuttgart / Online, 22-24 March 2021.
- L. Esguerra, L. Meßner, E. Robertson, N.V. Ewald, M. Gündoğan, and J. Wolters (2022). Optimization and readout-noise analysis of a hot vapor EIT memory on the Cs D1 line. Quantum Physics. https://doi.org/10.48550/arXiv.2203.06151 [Preprint]
- M. Yang, E. Robertson, L. Esguerra, K. Busch and J. Wolters (2023). Optical convolutional neural network with atomic nonlinearity. https://doi.org/10.48550/arXiv.2301.09994 [Preprint]
- L. Meßner, E. Robertson, L. Esguerra, K. Lüdge and J. Wolters (2023). Multiplexed random-access optical memory in warm cesium vapor. https://doi.org/10.48550/arXiv.2301.04885 [Preprint]
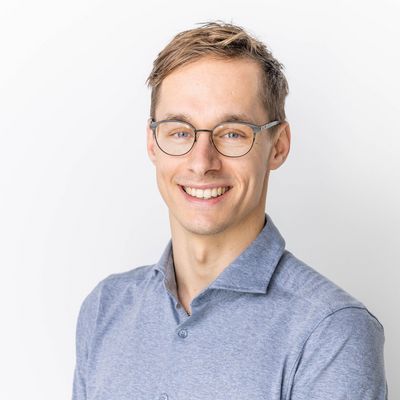
Jonas Schaible
HZB - FU Berlin
Contact
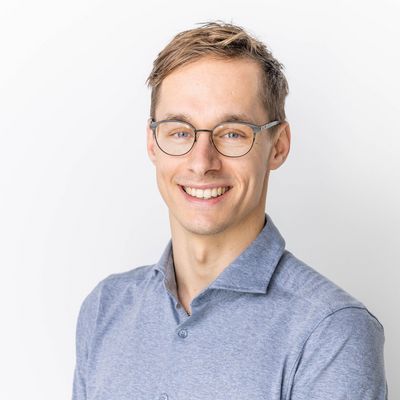
Supervisors:
With the growing share of photovoltaic (PV) solar energy in the global energy generation capacity, building-integrated photovoltaics (BIPV) gains increasing importance. For BIPV, aesthetical factors, such as color, play a larger role than for large industrial PV fields. The structural color of PV modules and surface texturing for minimizing reflective losses and for self-cleaning have to be considered for accurately estimating the optical performance and, subsequently, the annual energy yield. This raises several issues which are hardly considered in state-of-the-art numerical methods, which are used for planning PV systems and for estimating their performance: Which specific module surface treatments ensure maximum energy yield? How does the module appear visually to an observer throughout the day and year? How do the spectral albedo of the surroundings, local weather conditions and local shadowing affect the result? How can the complex environments in building-integrated and bifacial solar modules be simulated as efficiently as possible?
This project aims to develop an all-encompassing optical modeling and optimization toolbox for individual PV modules considering the full optical circumstances from specifically textured module surfaces to local solar irradiance conditions. Together with urban planners and architects, desirable color effects will be identified. Metrics will be developed in order to quantify the aesthetical effect of color appearance of BIPV modules. The PhD student will model PV modules with different textured surfaces and coloring techniques, and account for shadowing and spectral reflection of the surroundings, e.g. from overgrown ground and trees.
Full-length publications
- J. Schaible, H. Winarto, V. Škorjanc, D. Yoo, L. Zimmermann, K. Jäger, I. Sekulic, P.-I. Schneider, S. Burger, A. Wessels, B. Bläsi, and C. Becker (2024). Optimizing Aesthetic Appearance of Perovskite Solar Cells Using Color Filters. Solar RRL. https://doi.org/10.1002/solr.202400627
Conference presentations
- J. Schaible, B. Nouri, T. Kotzab, M. Loevenich, N. Blum, A. Hammer, K. Jäger, C. Becker and S. Wilbert. Application of Nowcasting to Reduce the Impact of Irradiance Ramps on PV Power Plants. (Oral presentation), EU PVSEC, Lisbon, Portugal, 18-22 September 2023.
- J. Schaible, H. Winarto, D. Yoo, L. Zimmermann, A. Wessels, K. Jäger, B. Bläsi, S. Burger, C. Becker. On aesthetical appearance of colored perovskite solar modules. (Oral presentation), SPIE Photonics Europe, Strasbourg, France, 8-12 April 2024.
- J. Schaible, H. Winarto, D. Yoo, L. Zimmermann, A. Wessels, K. Jäger, B. Bläsi, S. Burger, C. Becker. On aesthetical appearance of colored perovskite solar modules. (Poster presentation),16th Annual Meeting Photonic Devices (AMPD2024), Berlin, Germany, 17-19 April 2024.
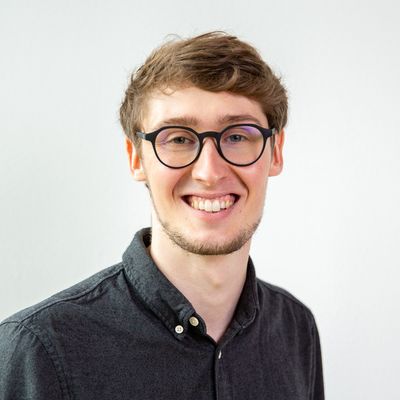
Hermann Stolte
HU Berlin - DESY
Contact
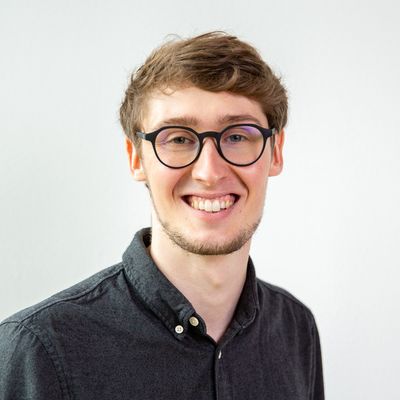
Supervisors:
The most exciting recent astrophysical events have involved transient phenomena. Notable examples are the possible correlation of an astrophysical neutrino with a flaring gamma-ray source, and the observation of gamma rays and gravitational waves produced by a Kilonova. The potential very-high-energy gamma-ray emission from such events is of particular scientific interest, tracking extreme acceleration processes. However, the occurrence of such transients can often only be anticipated on a very short term and, hence, is not considered in traditional approaches to scheduling observations.
This project sets out to increase the coverage of transient and variable gamma-ray sources through dynamic scheduling of observations. To this end, time series data representing light curves of gamma-ray and further energy bands will be used for short-term forecasting of flares, e.g., based on outlier detection or recurrent neural networks. Combining these forecasts with contextual information, such as monitored weather conditions, source positions in the sky, and telescope permutations in the participating array, the observing schedule is optimised.
Any realisation of the above idea, however, has to cope with scalability and responsiveness challenges: Time series data are collected at high rates (several hundred Hertz for current telescope arrays) and some gamma-ray sources are known to be variable at minute timescales. Hence, low-latency data processing and efficient online decision making are of crucial importance for dynamic observation scheduling. This project aims at providing the respective conceptual and technological foundations, by answering the following research questions:
• What are models for online decision making that combine approaches for flare forecasting with contextual information for optimal observation scheduling? This includes questions related to the expressiveness needed for the decision mechanism as well as the temporal and spatial granularity considered in these models.
• How can the online decision making be expressed in a computational model that is based on streaming data? Here, important aspects are the required operator algebra and data correlation mechanisms. Also, the notions of state to be maintained during processing is to be clarified.
• How to optimise the latency of stream processing for online decision making? Directions to answer this question are (i) prefetching of contextual information for low-latency assessment of flare forecasts, (ii) state management for streaming operators, and (iii) approximate stream processing using data sketches.
In sum, the results of this project will be a grounding of dynamic observation scheduling in models for data stream processing along with algorithm for their efficient realisation.
Full-length publications
- F. Schintke, K. Belhajjame, N.D. Mecquenem, D. Frantz, V.E. Guarino, M. Hilbrich, F. Lehmann, P. Missier, R. Sattler, J.A. Sparka, D. Speckhard, H. Stolte, A.D. Vu, and U. Leser (2024). Validity constraints for data analysis workflows. Future Generation Computer Systems, 157, 82-97. https://doi.org/10.1016/j.future.2024.03.037
- H. Stolte, J. Sinapius, I. Sadeh, E. Pueschel, M. Weidlich, and D. Berge (2025). Early Detection of Multiwavelength Blazar Variability. The Astrophysical Journal, 980, 1. https://doi.org/10.3847/1538-4357/ad960c
Conference presentations
- H. Stolte. Checking plausibility in exploratory data analysis. (Oral presentation), 47th International Conference on Very Large Databases, Copenhagen, Denmark, 16-20 August 2021. http://ceur-ws.org/Vol-2971/paper08.pdf
- H. Stolte, J. Sinapius, I. Sadeh, E. Pueschel, D. Berge, and M. Weidlich. Detecting VHE blazar flares with deep learning. (Oral presentation), International Conference on Machine Learning for Astrophysics - ML4Astro, Catania, Italy, 30 May - 02 June 2022.
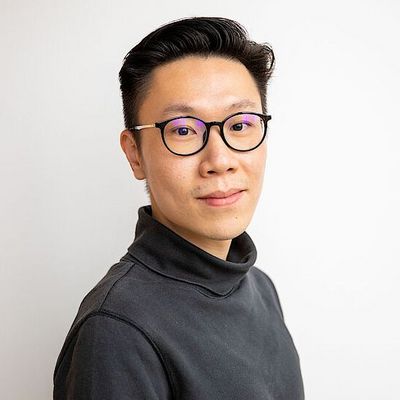
Christian Utama
FU Berlin - HZB
Contact
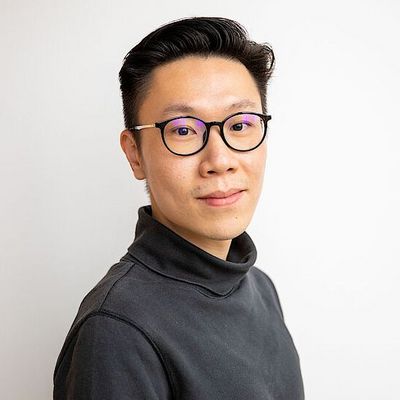
Supervisors:
Today, because of the complexity of underlying machine learning models, AI appears as a ‘black box’, since the internal learning and optimization processes are often not completely comprehensible. To tackle the trade-off problem, methods of “Explainable Artificial Intelligence” (XAI) were developed to increase the transparency of underlying models without decreasing their performance. In this context, the PhD project aims to apply XAI methods for machine learning models in two use cases in the field of energy, esp. photovoltaics, to increase the models’ explainability and hence trustworthiness.
The first use case is linked to the existing HEIBRiDS project “Optimization of solar energy yield..” The control strategies in this project incorporate predictive and prescriptive data analytics based on machine learning approaches, which however represent black boxes. The XAI research proposed here makes it possible both to improve the trust and acceptability of AI-based solutions, and to generate findings that sustainably improve product design or system configurations. The second use case relates to the “Combinatorial materials discovery” pursued in the HZB research groups Unold/Schorr and Abdi/van de Krol, which focuses on the exploration of light-absorbing semiconductor sand catalysts for solar energy conversion devices by combinatorial high throughput methods. This generates high-dimensional sets of data, which have to be searched and analyzed for structure-property-function relationships and from which guidance for further experiments is sought. For this purpose, it is aimed to use machine learning approaches to automate time-consuming procedures regarding the analysis of the multidimensional datasets. The introduction of machine learning in combination with XAI methods is hence expected to accelerate development processes, to provide new physical understandings and eventually support the efficient development of materials for solar conversion devices.
The results of the PhD project will be synthesized to contribute knowledge regarding a) the validity and reliability of XAI methods in the field of photovoltaic, b) new opportunities for data pre-processing and data analytics based on XAI outcomes, c) consequent effects on the development of (and trust towards) energy systems, and d) the findings’ transferability to other fields in the energy sector.
Full-length publications
- C. Utama, C. Meske, J. Schneider, and C. Ulbrich (2022). Reactive power control in photovoltaic systems through (explainable) artificial intelligence. Applied Energy, 328. https://doi.org/10.1016/j.apenergy.2022.120004
- C. Utama, B. Karg, C. Meske, and S. Lucia (2022). Explainable artificial intelligence for deep learning-based model predictive controllers. In Proceedings of the 26th International Conference on System Theory, Control and Computing (ICSTCC), 464-471. https://doi.org/10.1109/ICSTCC55426.2022.9931794
- C. Utama, C. Meske, J. Schneider, R. Schlatmann, and C. Ulbrich (2023). Explainable artificial intelligence for photovoltaic fault detection: A comparison of instruments. Solar Energy, 249, 139–151. https://doi.org/10.1016/j.solener.2022.11.018
Conference presentations
- C. Utama, C. Meske, J. Schneider, and C. Ulbrich. Reactive power control in photovoltaic systems through (explainable) artificial intelligence. (Poster presentation), 8th World Conference on Photovoltaic Energy Conversion (WCPEC-8), Milan, Italy, 26-30 September 2022.
- C. Utama, B. Karg, C. Meske, and S. Lucia. Explainable artificial intelligence for deep learning-based model predictive controllers. (Oral presentation), 26th International Conference on System Theory, Control and Computing (ICSTCC), Online, 19-21 October 2022.
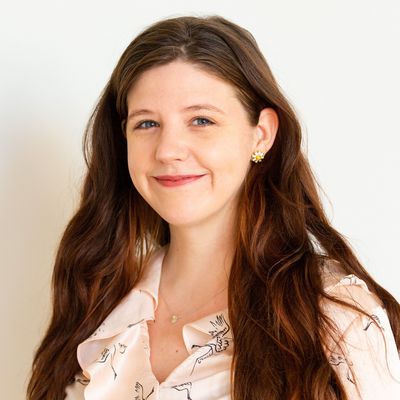
Femke van Geffen
AWI - TU Berlin
Contact
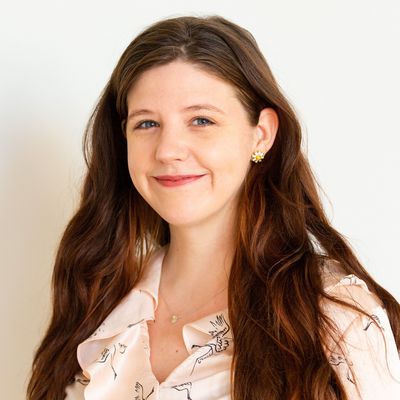
Supervisors:
The Polar Terrestrial Environmental Systems Research Group at the Alfred-Wegener-Institut has multiple field trips a year that, among other goals, aim to collect data to monitor the landcover and vegetation dynamics in the Arctic region. Vegetation dynamics can provide an insight into the effects of global warming on the environment. The aim of the current project is to employ Machine Learning and Deep learning methods to analyse this data to gain better insights into the dynamics of the vegetation species and how these are changing over time. In order to accomplish this goal, various types of Remote Sensing data is used such as Sentinel-2, Landsat 7/8 as well as drone data collected in the field. The ultimate goal is to develop a fusion method that can utilise the available data to create a comprehensive overview of vegetation dynamics of the past, present and future.
Full-length publications
- F. van Geffen, B. Heim, F. Brieger, ..., U. Herzschuh, and S. Kruse. SiDroForest: A comprehensive forest inventory of Siberian boreal forest investigations including drone-based point clouds, individually labelled trees, synthetically generated tree crowns and Sentinel-2 labelled image patches. Earth Syst. Sci. Data, 14, 4967–4994, 2022. https://doi.org/10.5194/essd-14-4967-2022
Conference presentations
- F. van Geffen, B. Heim, U. Herzschuh, L. Pestryakova, E. Zakharov, R. Hänsch, B. Demir, B. Kleinschmit, M.Förster, and S. Kruse. SiDro Forest: Siberian drone-mapped forest inventory. (Oral presentation), Arctic Science Summit Week, Lisbon, Portugal / Online,19-26 March 2021.
- F. van Geffen, B. Heim, U. Herzschuh, L. Pestryakova, E. Zakharov, R. Hänsch, B. Demir, B. Kleinschmit, M.Förster, and S. Kruse. SiDro Forest: Siberian drone-mapped forest inventory. (PICO presentation), EGU General Assembly, Online, 19-30 April 2021. https://doi.org/10.5194/egusphere-egu21-15106
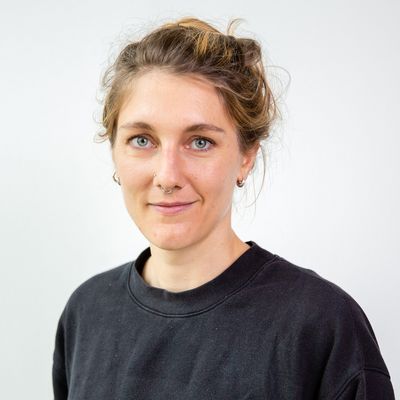
Nadja Veigel
TU Berlin - GFZ
Contact
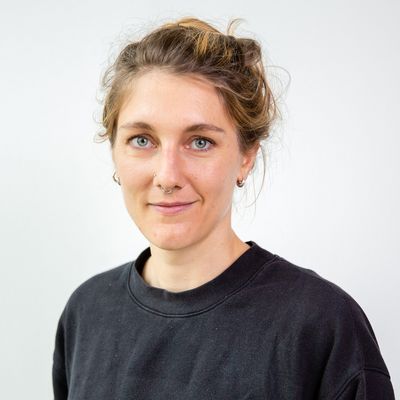
Supervisors:
Flooding events in urban and non-urban settings represent a major cause of insured losses, with costs of several billion US$/year globally (e.g., US$ 60 billion in 2016). Both people and infrastructural assets are vulnerable to flood events, which are becoming increasingly frequent and severe as a consequence of climate change, extreme rainfall events, and the increasing number of people living in flood-prone areas. Several quantitative approaches for flood risk assessment exist in the literature. They rely on statistical methods and hydrological models to quantify the expected risk as a function of hazard (i.e., flood extent depth), exposure (of people/assets), and vulnerability (damage). Yet, there is limited integration of dynamic human behaviors in such methods. Human behavior dynamics play a key role in affecting the impact and recovery time of floods in coupled human-environment systems. The perception of risk, as well as adaptive behaviors for prevention, preparation, response, and recovery during flood events (e.g., accessing weather warnings, donating money for recovery) depend on several individual and collective socio-psychographic determinants. One of the key challenges in quantitative risk assessment at present is how to integrate information on dynamic human behaviors in risk assessment models. These should then inform precautionary and emergency measures and risk management approaches which mitigate flood risk.
This project addresses the above challenge as articulated in these three specific questions: (i) which data and approaches can be utilized to better understand and model relevant human behaviors before, during, and after flood events? (ii) how can relevant human behaviors be learned from the above data and integrated in dynamic flood risk assessments to support decision-making in risk management and climate adaptation? (iii) which environmental and economic benefits can be achieved by embedding human behaviors in flood risk models?
Full-length publications
- N. Veigel, H. Kreibich, and A. Cominola (2022). A gradient boosting approach to identify behavioral and policy determinants of flood resilience in the continental US. IFAC-PapersOnLine, 55, 33, 85–91. https://doi.org/10.1016/j.ifacol.2022.11.014
- N. Veigel, H. Kreibich, and A. Cominola (2023). Interpretable machine learning reveals potential to overcome reactive flood adaptation in the continental US. Earth's Future, 11, e2023EF003571. https://doi.org/10.1029/2023EF003571
Conference presentations
- N. Veigel, H. Kreibich, and A. Cominola. Mining flood insurance big data to reveal the determinants of humans' flood resilience. (Oral presentation), EGU General Assembly, Online, 19–30 Apr 2021. https://doi.org/10.5194/egusphere-egu21-3042
- N. Veigel, H. Kreibich, and A. Cominola. Mining flood insurance big data to incorporate behavioural and social aspects in flood risk modelling (Poster presentation), AGU Fall Meeting, Online & New Orleans, USA, 13–17 Dec 2021, SY55D-0390.
- N. Veigel, H. Kreibich, and A. Cominola. Exploring Behavioral Determinants of Flood Insurance Adoption with Explainable Machine Learning in the Continental US. EGU General Assembly, Vienna, Austria & Online, 23-27 May 2022. https://doi.org/10.5194/egusphere-egu22-5839
- N. Veigel, H. Kreibich, J.A. de Bruijn, J.C.J.H. Aerts, and A. Cominola. A Transformer-Based Analysis of Tweets in Germany to Investigate the Appearance and Evolution of the 2021 Eifel Flood in Social Media. (Poster presentation), EGU General Assembly, Vienna, Austria, 24–28 April 2023. https://doi.org/10.5194/egusphere-egu23-6038
- N. Veigel, H. Kreibich, J. de Bruijn, J.C.J.H. Aerts, and A. Cominola. Correlation of Social Media-Driven Risk Perception and Flood Insurance Uptake for Floods in the US. EGU General Assembly, Vienna, Austria, 14–19 April 2024. https://doi.org/10.5194/egusphere-egu24-11646
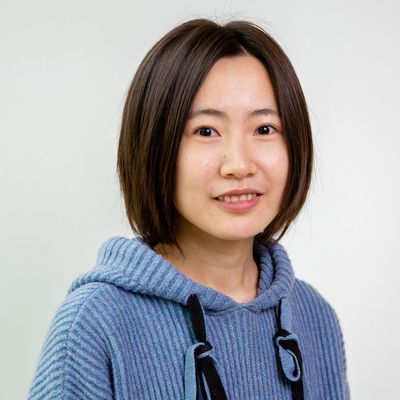
Xiaoyan Yu
MDC - Charité - Uni Potsdam
Contact
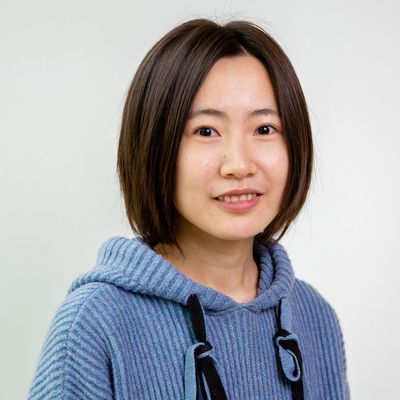
Supervisors:
The field of digital pathology is growing and people have higher and higher expectations of artificial intelligence to realize the automatic diagnosis. Before achieving automatic medicine diagnosis or other specific clinic tasks, realizing medical images semantic instance segmentation, which is pixel-wise recognition of objects and their classification into meaningful categories, is a key step. And semantic instance segmentation is also a classical task in the computer vision area. The great success of semantic instance segmentation by applying neural networks has shown on nature images with the help of a large amount of annotated dataset. While this can be achieved for microscope images, there are problems: new types of samples by means of microscopy are continually being entered into medical image datasets and getting annotated samples of each type for training neural networks would not be feasible. Secondly, diverse microscopy modality including upcoming techniques is being developed which makes this task even more complex. To date, there are no automated methods that are able to perform desired image analyses at the necessary level of accuracy without extensive manual input. This particularly holds for microscopy data of cells in heterogeneous tissue, where the cost for accurate outlining of cell boundaries, be it as part of a manual analysis or as part of generating training data for deep learning methods, restricts the feasibility of high-content studies.
In this project, we aim at overcoming this restriction by leveraging “sparse” annotations for training deep neural networks for semantic instance segmentation, which amounts to the weak supervision. We will develop a model for learning pixel-accurate instance segmentation purely from center point annotations, which is an unsolved problem for clusters of densely packed objects, like cells in tissue. Beyond leveraging center point annotations, we will investigate alternative sparse annotations, like image-level labels, in terms of their potential to be generated by crowd workers. In the extreme case, we want to explore the possibility of solving the problem in an unsupervised manner. We would like to investigate on a deep learning method applied to medical images doing semantic instance segmentation task without any labels and its limit.
Full-length publications
- J.L. Rumberger, X. Yu, P. Hirsch, M. Dohmen, V.E. Guarino, A. Mokarian, L. Mais, J. Funke, and D. Kainmueller (2021). How shift equivariance impacts metric learning for instance segmentation. Proceedings of the IEEE/CVF International Conference on Computer Vision (ICCV).
Conference presentations
-