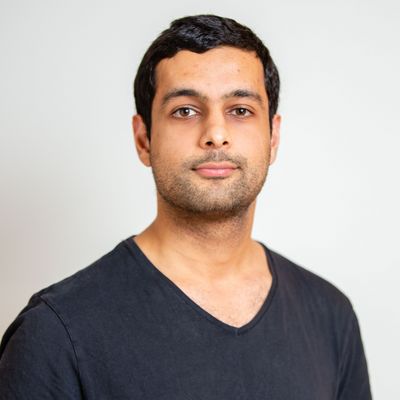
Siddhant Agarwal
DLR - TU Berlin
Contact
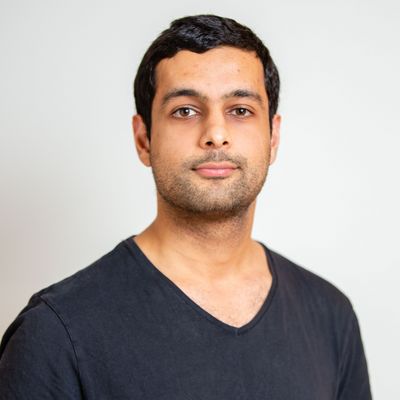
Supervisors:
Studying how rocky planets like Mercury, Venus, the Earth and Mars evolve over billions of years requires detailed modelling of mantle convection, the main driver of planetary evolution. The mantle - sandwiched between the crust and the core - behaves like a highly viscous fluid over geological time scales and hence can be quantified through equations describing conservation of mass, momentum and energy. These non-linear partial differential equations are typically solved numerically using fluid dynamics codes. However, the parameters and initial conditions to these equations are poorly known. Whereas certain outputs of the simulations (numerically solved equations) can be "observed'' via spacecraft missions and used to constrain key parameters and initial conditions, thus elucidating the basic physics and evolution of planets. Since each simulation can take from several hours to weeks to run, varying parameters extensively and repeatedly is often impractical. We aim to overcome this computational bottleneck by learning the mapping between parameters and observables through a combination of state-of-the-art geodynamic modelling, machine learning and high-performance computing.
Doctoral thesis
S. Agarwal (2022). Unraveling the interior evolution of terrestrial planets through machine learning. Technische Universität zu Berlin.
doi:10.14279/depositonce-15926
Full-length publications
- S. Agarwal, N. Tosi, D. Breuer, S. Padovan, P. Kessel, and G. Montavon (2020). A machine-learning-based surrogate model of Mars’ thermal evolution. Geophysical Journal International, 222(3), 1656-1670. https://doi.org/10.1093/gji/ggaa234
- S. Agarwal, N. Tosi, P. Kessel, S. Padovan, D. Breuer, and G. Montavon (2021). Towards constraining Mars’ thermal evolution using Machine Learning. Earth and Space Science.https://doi.org/10.1029/2020EA001484
- S. Agarwal, N. Tosi, P. Kessel, D. Breuer, and G. Montavon (2021). Deep learning for surrogate modeling of two-dimensional mantle convection. Physical Review Fluids, 6, 113801. https://doi.org/10.1103/PhysRevFluids.6.113801
Conference presentations
- S. Agarwal, N. Tosi, D. Breuer, S. Padovan, P. Kessel, and G. Montavon. Unravelling interior evolution of terrestrial planets using Machine Learning. (Oral presentation), Artificial Intelligence in Astronomy at ESO, Garching, Germany, 22-26 July 2019.
- S. Agarwal, N. Tosi, D. Breuer, P. Kessel, and G. Montavon. Using machine learning to predict 1D steady-state temperature profiles from compressible mantle convection simulations. (Oral presentation), 72nd Annual Meeting of the APS Division of Fluid Dynamics, Seattle, USA, 23-26 November 2019.
- S. Agarwal, N. Tosi, P. Kessel, D. Breuer, S. Padovan, and G. Montavon. Mars’ thermal evolution from machine-learning-based 1D surrogate modelling. (Oral presentation), EGU General Assembly, Online, 7 May 2020.
- S. Agarwal, N. Tosi, P. Kessel, D. Breuer, S. Padovan, and G. Montavon. Learning high dimensional surrogates from mantle convection simulations. (Oral presentation), 73rd Annual Meeting of the APS Division of Fluid Dynamics, Online, 23 November 2020.
- S. Agarwal, N. Tosi, P. Kessel, S. Padovan, D. Breuer, and G. Montavon. Towards constraining Mars' thermal evolution using machine learning. (PICO presentation), EGU General Assembly, Online, 19-30 Apr 2021. https://doi.org/10.5194/egusphere-egu21-4044
- S. Agarwal, N. Tosi, P. Kessel, D. Breuer, and G. Montavon. Deep learning for surrogate modelling of 2D mantle convection, oral presentation. German-Swiss Geodynamics Workshop 2021, Bad Belzig, 29 Aug–1 Sep 2021.
- S. Agarwal, N. Tosi, P. Kessel, D. Breuer, and G. Montavon. Deep learning for surrogate modelling of 2D mantle convection. (Oral presentation), European Planetary Science Congress 2021, Online, 13–24 Sep 2021. https://doi.org/10.5194/epsc2021-218
- S. Agarwal, N. Tosi, P. Kessel, D. Breuer, and G. Montavon. Deep learning for surrogate modelling of 2D mantle convection. (Oral presentation), The 74th Annual Meeting of the Division of Fluid Dynamics, Online, 21-23 Nov 2021.
- S. Agarwal, N. Tosi, P. Kessel, D. Breuer, and G. Montavon. A machine learning framework for constraining mantle convection parameters. (Oral presentation), American Geophysical Union Fall Meeting, New Orleans, 13-17 Dec 2021.
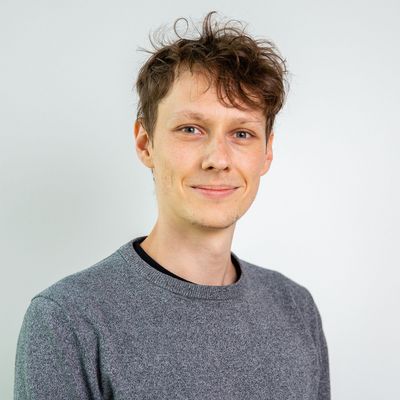
Philipp Baumeister
DLR - TU Berlin
Contact
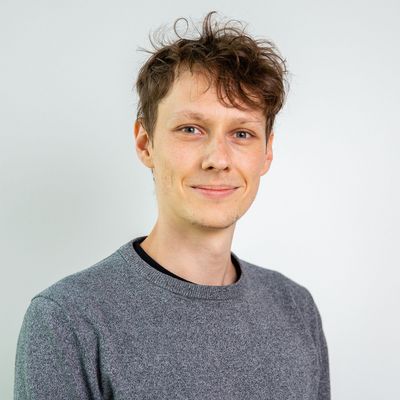
Associated Doctoral Researcher
Supervisors:
Since the first discoveries of extrasolar planets in the 1990s, more than 4000 exoplanets have been discovered to date, and the number is growing rapidly with new dedicated space and ground-based surveys. From radius measurements via transit observations and mass estimations via radial velocity measurements, the inner structure of planets can be modeled numerically. This characterization is crucial for our understanding of the diversity of the observed planets, their formation processes, and the question whether or not they can support life. However, even with accurate radius and mass measurements, many different solutions for the internal structure can be found, since the relative proportions of iron, silicates, water ice, and volatile elements are not known.
The goal of this project is to implement machine-learning-based approaches to infer planetary interiors based on observational data, and use those to identify potentially observable parameters that can better constrain the range of possible interior structures. Machine learning can avoid the need for extensive interior modeling for each individual exoplanet by learning from large sets of precalculated data generated with suitable forward models. We aim to develop such an inference framework for the fast characterization of planetary interiors. For a comprehensive view of a planet's evolution, we will link thermal evolution models of the interior to models of atmospheric evolution, and aim at including results from population synthesis modeling. These models contain essential information on the structure, composition and evolution history of planets, linking the planet interior to the star system they reside in, and supply us with a large data set of synthetic planets that have formed under the physical constraints of their formation model.
The result of this project will be a comprehensive inference model capable of rapidly determining the range of physically meaningful interiors of observed exoplanets, which will open up new possibilities for finding observable parameters that are particularly important in constraining possible internal structures.
Doctoral thesis
P. Baumeister (2023). Interior structure, mantle-atmosphere co-evolution, and habitability of low-mass exoplanets. Technische Universität zu Berlin. doi.org/10.14279/depositonce-19452
Full-length publications
- P. Baumeister, S. Padovan, N. Tosi, G. Montavon, N. Nettelmann, J. MacKenzie, and M. Godolt (2020). Machine-learning Inference of the Interior Structure of Low-mass Exoplanets. Astrophysical Journal, 889, 42. https://doi.org/10.3847/1538-4357/ab5d32
- S. Padovan, T. Spohn, P. Baumeister, N. Tosi, D. Breuer, S. Csizmadia, H. Hellard and F. Sohl (2018). Matrix-propagator approach to compute fluid Love numbers and applicability to extrasolar planets. Astronomy & Astrophysics, 620, A178. https://doi.org/10.1051/0004-6361/201834181
Conference presentations
- P. Baumeister, S. Padovan, N. Tosi, G. Montavon, N. Nettelmann, J. MacKenzie, and M. Godolt. Machine-learning inference of the interior structure of low-mass exoplanets. (Oral presentation), EGU General Assembly 2020, Vienna, Austria, 4 - 8 May 2020.
- P. Baumeister, S. Padovan, N. Tosi, G. Montavon, N. Nettelmann, J. MacKenzie and M. Godolt. Using machine learning to infer the interior structure of exoplanets. (Oral presentation), EPSC-DPS Joint Meeting 2019, Geneva, Switzerland, 15 - 20 September 2019.
- P. Baumeister, S. Padovan, N. Tosi, G. Montavon, J. MacKenzie and M. Godolt. Using mixture density networks to infer the interior structure of exoplanets. (Poster presentation), Artificial Intelligence in Astronomy Workshop, ESO, Garching, Germany, 22 - 26 July 2019.
- P. Baumeister, S. Padovan, N. Tosi, G. Montavon. Using deep learning neural networks to predict the interior composition of exoplanets. (Poster presentation), PLATO Theory Workshop 2018, Cambridge, UK, 3 - 5 December 2018.
- P. Baumeister, J. MacKenzie, N. Tosi, and M. Godolt. Effects of different equations of state on interior structure models of exoplanets. (Oral presentation), 7th Joint Workshop on High Pressure, Planetary and Plasma Physics (HP4), Berlin, Germany, 10 - 12 October 2018.
- P. Baumeister, J. MacKenzie, N. Tosi, and M. Godolt. Effects of different equations of state on interior structure models of exoplanets. (Oral presentation), European Planetary Science Congress 2018, Berlin, Germany, 16 - 21 September 2018.
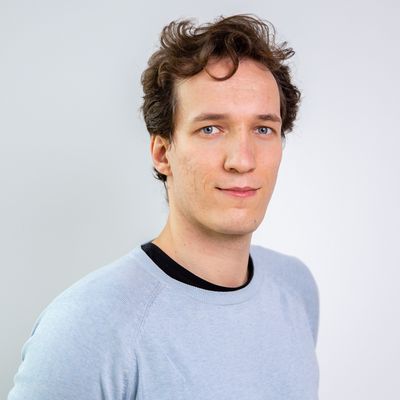
Ivo Daniel
TU -Berlin
Contact
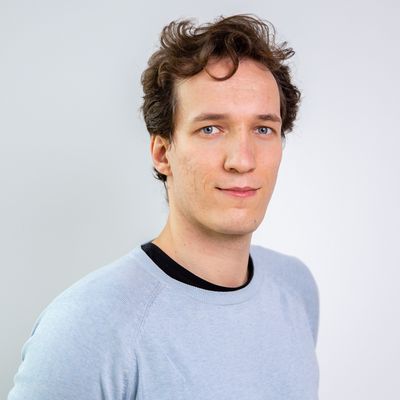
Associated Doctoral Researcher
Supervisors:
Water losses are one of the main consequences of infrastructure failures in water distribution networks. While background leakages and pipe bursts in well maintained systems generally amount to only 3-7% of the total water supplied, they can account for more than 50% for poorly maintained networks worldwide. Methods that support prompt detection and accurate localization of leakages are crucial to help water utilities implement timely mitigation measures and avoid unnecessary loss of water.
Leakages can be classified as one type of anomaly occurring in water distribution networks. Broadly speaking, methods for their detection are referred to as anomaly detection methods. Anomaly detection methods have been studied extensively in the context of intrusion into information networks, and applied to water distribution networks in the similar context of cyber-attacks on SCADA systems. However, most current approaches for leakage detection rely on in-situ, engineering-based technology, while the development and application of data-driven approaches still poses several research challenges.
The goal of this project is to develop data-driven methods that are capable of detecting leakages in water distribution networks in real-time. As this research originated in an international competition, the BattLeDIM - Battle on Leakage Detection and Isolation Methods (http://battledim.ucy.ac.cy), its foundation is built upon the BattLeDIM dataset, inferring that the focus is put on the analysis of high resolution pressure data provided by a network of sensors located throughout the system. Data Mining and Machine Learning frameworks offer a wide range of opportunities for the analysis of this data and are comparatively utilized to identify and localize leakages as the primary type of anomaly.
The development of a data-driven methodology for leakage detection opens up the possibility to be extended to other applications in water distribution systems, including real-world systems, and assess their transferability to other problems where anomaly detection may be beneficial. The development of such an effective, decentralized framework implies the opportunity for additional research on IoT sensors, their communication interface, and their placement. Further research may be targeting wastewater systems to evaluate whether the developed methods may be cost-effectively transferred or adapted.
Doctoral thesis
I. Daniel (2023). Physics-Informed Anomaly Detection in Water Distribution Systems - Advancing Digital Transformation of Urban Water Management. Technische Universität zu Berlin. doi:10.14279/depositonce-19551
Full-length publications
- I. Daniel, J. Pesantez, S. Letzgus, M.A. Khaksar Fasee, F. Alghamdi, E. Berglund, G. Mahinthakumar, and A. Cominola (2022). A sequential pressure-based algorithm for data-driven leakage identification and model-based localization in water distribution networks. Journal of Water Resources Planning and Management, 148, 6. DOI:10.1061/(ASCE)WR.1943-5452.0001535
- I. Daniel, N.K. Ajami, A. Castelletti, D. Savic, R.A. Stewart, and A. Cominola (2023). A survey of water utilities’ digital transformation: drivers, impacts, and enabling technologies. npj Clean Water, 6, 51. https://doi.org/10.1038/s41545-023-00265-7
- I. Daniel, G.R. Abhijith, L. Kadinski, A. Ostfeld, and A. Cominola, A. (2023). A Machine Learning-Based Surrogate Model for Coupled Hydraulic and Water Quality Simulation in Water Distribution Networks. Proceedings of the World Environmental and Water Resources Congress, 817–830. https://ascelibrary.org/doi/10.1061/9780784484852.077
- I. Daniel, and A. Cominola (2023). Estimating irregular water demands with physics-informed machine learning to inform leakage detection. arXiv. https://doi.org/10.48550/ARXIV.2309.02935 [Preprint]
Conference presentations
- I. Daniel, N. Ajami, A. Castelletti, D. Savic, R. Stewart, M. Becker, and A. Cominola. How is digital transformation impacting the water utility sector? - Insights from a worldwide online utility survey. (Oral presentation), EGU General Assembly 2021, Online, 19–30 Apr 2021. https://doi.org/10.5194/egusphere-egu21-12540
- I. Daniel, J. Pesantez, S. Letzgus, M.A. Khaksar Fasee, F. Alghamdi, E. Berglund, G. Mahinthakumar, and A. Cominola. Leakage identification and localization on the BattLeDIM dataset: testing and performance evaluation of a high-resolution pressure-driven method. (Oral presentation), World Environmental & Water Resources Congress, Online, 7-11 Jun 2021.
- G. Pedron, I. Daniel, D. Tilcher, A. Cominola and A. Crescenti. Gaza H2.0: promoting sustainable water supply and demand and knowledge transfer to enhance water infrastructure resilience in the Gaza Strip. (Oral presentation), 42nd WEDC International Conference, online, 13-15 September 2021. https://hdl.handle.net/2134/16903540.v1
- I. Daniel, N. Ajami, A. Castelletti, D. Savic, R. Stewart, and A. Cominola. How Is Digital Transformation Impacting The Water Utility Sector? Insights From A Worldwide Online Utility Survey. (Oral presentation), IWA World Water Congress & Exhibtion, Copenhagen, 11-15 September 2022.
- I. Daniel, and A. Cominola. A calibration-free pressure-driven approach to leak detection and localization in water distribution networks. (Oral presentation), World Environmental & Water Resources Congress, Henderson, NV, USA, 21-24 May 2023.
- I. Daniel, and A. Cominola. Physics-Informed Neural Networks to enhance leakage detection in drinking water distribution systems. (Oral presentation), EGU General Assembly, Vienna, Austria, 24-28 April 2023. https://doi.org/10.5194/egusphere-egu23-12186
- A. Cominola, I. Daniel, D. Tilcher, A.J.S. Alasmar, R.M.M. Ziara, and G. Pedron. Enhancing the resilience of intermittent water supply systems in Khan Younis, Gaza Strip. Knowledge transfer and lessons learned from the Gaza H2.0 project. (Oral presentation), EGU General Assembly, Vienna, Austria, 24-28 April 2023. https://doi.org/10.5194/egusphere-egu23-13100
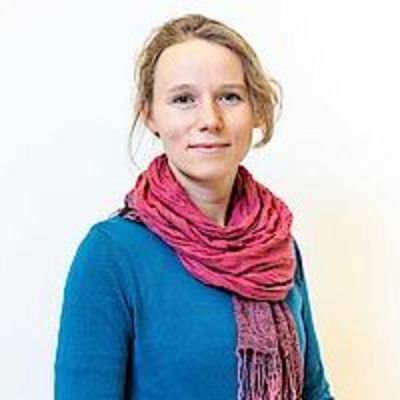
Veronika Doepper
AWI - TU Berlin
Contact
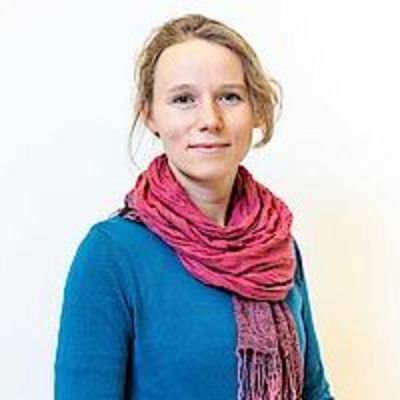
(Interrupted studies before PhD degree)
Supervisors:
Our goal is to employ vomputer vision and data science methods to advance the data handling, analyses and interpretation of the wealth of Big Data 3-D remotely sensed environmental data acquired by the AWI on polar expeditions. Objective: Data acquisitions from drone-borne and airplane passive and active optical imaging sensors over large areas in Siberia and Alaska resulted in multi-temporal datasets of billions of remotely sensed points in spatially explicit point clouds. Computer vision already enables notable advancements in 2-D Big Data environmental data science. In addition, a wide range of 3-D sensors is employed to investigate polar terrestrial environments and permafrost landscapes: ground-based, drone-borne, and airplane active optical laser scanning devices and passive optical densely overlapping imaging from different view angles provide 3-D point cloud data consisting of billions of individual measurements of surface structures, including vegetation and permafrost landscape topography in the circumpolar region. Approach: 3-D point clouds containing billions of remotely sensed points over large areas are the products from the drone-borne and airplane LIDAR as well as high-resolution Red Green Blue and Red Green Near Infrared cameras that allow stereo photogrammetric derivation of point clouds. We need machine learning on the Big Data 3-D point clouds that will also allow us to analyse the characteristics and interactions among the points in 3-D space to enable recognition of tree species and terrestrial degradation features (classification), to enable segmentation to identify the meaning of the environmental objects, and to develop advanced automated change detection tools for multi-temporal point cloud datasets. We will apply two use cases using high latitude biodiversity and permafrost landscape diversity.
Full-length publications
-
Conference presentations
- V. Döpper, R. Jackisch, J. Gloy, T. Rettelbach, J. Boike, …, G. Grosse, and S. Kruse. Towards an automatic segmentation and classification of multi-source point clouds for Arctic to boreal permafrost ecosystem analysis. (Poster presentation), EGU General Assembly, Vienna, Austria, 24–28 April, 2023. https://doi.org/10.5194/egusphere-egu23-15600
- V. Döpper et al. Unlocking the Potential of Arctic to Boreal Multi-Source Point Clouds: Deep and Transfer Learning for Automated Segmentation and Classification. (Poster presentation), SilviLaser 2023, London, UK, 6-8 September, 2023.
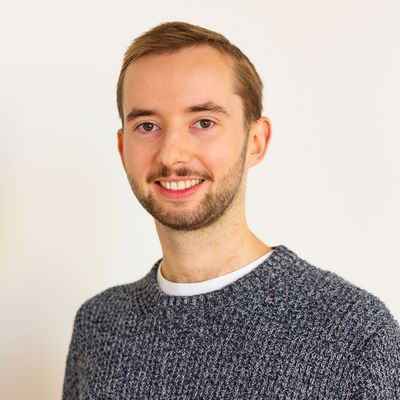
Felix Fiedler
TU-Dortmund
Contact
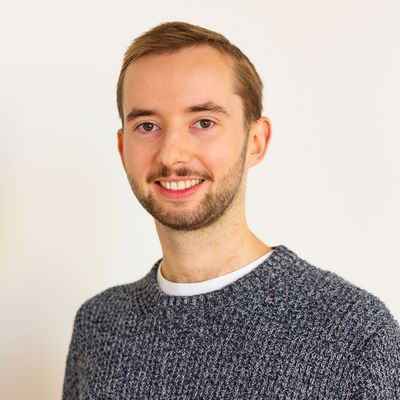
Associated Doctoral Researcher
Supervisors:
Recent advances in ultra-low-power microcontrollers and FPGAs together with the possibility of tailoring optimization algorithms and new machine learning techniques to such hardware make it possible to perform, on the edge, complex data analytics that were previously only possible on powerful computers. These techniques are especially relevant in applications such as planetary exploration missions where communication is not available in real-time and all computations should occur on-board. This project focuses on the following three areas:
Development of novel methods for embedded data analytics: Many applications in the space sciences or the internet of Things require the use of low-power devices. New research will be performed to develop new algorithms for the solution of optimization problems and machine learning techniques that are tailored to new hardware architectures. In particular, ultra-low-power microcontrollers and FPGAs will be studied.
Low-power and energy-aware data analytics: a co-design of the developed algorithms will be performed by analyzing performance and energy consumption. The goal is to provide optimal tradeoffs between performance and energy consumption, which can be adapted according to the current energy availability in different applications. Self-localization systems: when satellite-based systems are not available, being able to perform autolocalization is a critical task to any tasks that requires autonomous decision making as in planetary exploration missions. The developed methods will be applied and tailored for the challenging tasks usually encountered in self-localization systems for exploration missions.
Doctoral thesis
F. Fiedler, S. Lucia, and S. Gros (2024) Data-based predictive control with nonlinear and multi-step models. Universitätsbibliothek Dortmund. https://doi.org/10.17877/de290r-24552.
Full-length publications
- F. Fiedler, C. Dopmann, F. Tschorsch, and S. Lucia (2020). PredicTor: Predictive congestion control for the Tor network.IEEE Conference on Control Technology and Applications (CCTA), 863-870. 10.1109/ccta41146.2020.9206384
- F. Fiedler, D. Baumbach, A. Borner, and S. Lucia (2020). A probabilistic moving horizon estimation framework applied to the visual-inertial sensor fusion problem. European Control Conference (ECC), 1009-1016. 10.23919/ecc51009.2020.9143645
- P. Guillen, F. Fiedler, H. Sarnago, S. Lucia, O. Lucia, and S. Lucia. (2022). Deep learning implementation of model predictive control for multioutput resonant converters. IEEE Access, 10, 6522865237.
- C. Döpmann, F. Fiedler, S. Lucia, and F. Tschorsch (2022). Optimization-based predictive congestion control for the Tor Network: Opportunities and challenges. ACM Transactions on Internet Technology, 22, 4, 130.
Conference presentations
-
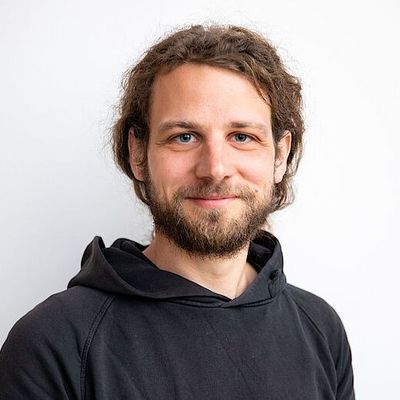
Peter Hirsch
MDC
Contact
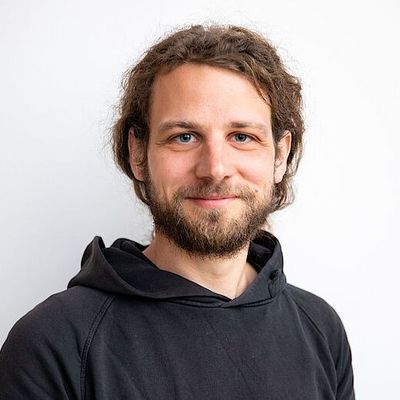
Associated Doctoral Researcher
Supervisors:
Many experiments in biology require a large number of samples to allow for conclusive statements. It is thus of utmost importance to reduce the cost per sample as much as possible. This cost can be both in terms of money and time. If a single sample takes multiple hours and one needs hundreds or thousands of samples, the undertaking quickly becomes infeasible. Every significant reduction in manual work can thus make an infeasible project feasible.
In this project we want to study the effect of changes (mutations) in the genome - some of them lethal - on the development of C. elegans embryos and its cell lineage. Yet this requires the tracking of all their cells over time and through cell divisions. While some automatic methods to do this exist, all require several hours of manual curation per sample to get an error-free result.
To overcome this, we are developing new tracking algorithms employing modern machine learning methods applied to volumetric time series data (3d+time).
C. elegans provides us with a prime example. Its development is stereotypical, each wild type (without mutations) organism exhibits the identical number of cells and division pattern. This makes it possible to automatically pin-point both errors in the tracking algorithm and true changes in the development due to mutations.
Analyzing these changes will help us to expand our understanding of the gene regulatory networks induced by the genome, and how they are affected by mutations, a key challenge of developmental biology.
Doctoral thesis
P.J. Hirsch (2023). Segmentation and Tracking of Cells and Nuclei Using Deep Learning. Humboldt Universität. https://doi.org/10.18452/26934
Full-length publications
- A. Krull*, P. Hirsch*, C. Rother, A. Schiffrin, and C. Krull (2020). Artificial-intelligence-driven scanning probe microscopy. (*shared first) Commun Phys 3, 54. https://doi.org/10.1038/s42005-020-0317-3
- P. Hirsch, and D. Kainmueller (2020). An auxiliary task for learning nuclei segmentation in 3D microscopy images. Proceedings of Machine Learning Research 121(304), 318.
- L. Mais*, P. Hirsch*, and D. Kainmueller (2020). PatchPerPix for instance segmentation. (*shared first) In: Vedaldi A., Bischof H., Brox T., Frahm JM. (eds) Computer Vision – ECCV 2020. ECCV 2020. Lecture Notes in Computer Science, vol 12370. Springer, Cham. https://doi.org/10.1007/978-3-030-58595-2_18
- J.L. Rumberger*, X. Yu*, P. Hirsch*, M. Dohmen*, V.E. Guarino*, A. Mokarian, L. Mais, J. Funke, and D. Kainmueller (2021). How Shift equivariance impacts metric learning for instance segmentation. (*shared first) In Proceedings of the IEEE/CVF International Conference on Computer Vision (ICCV).
-
L. Mais, P. Hirsch, C. Managan, K. Wang, K. Rokicki, R.R. Svirskas, B.J. Dickson, W. Korff, G.M. Rubin, G. Ihrke, G.W. Meissner, and D. Kainmueller (2021). PatchPerPixMatch for Automated 3d Search of Neuronal Morphologies in Light Microscopy. bioRxiv. https://doi.org/10.1101/2021.07.23.453511
- P. Hirsch, C. Malin-Mayor, A. Santella, S. Preibisch, D. Kainmueller, and J. Funke (2022). Tracking by Weakly-Supervised Learning and Graph Optimization for Whole-Embryo C. elegans lineages. In: Wang, L., Dou, Q., Fletcher, P.T., Speidel, S., Li, S. (eds) Medical Image Computing and Computer Assisted Intervention – MICCAI 2022. Lecture Notes in Computer Science, vol 13434. Springer, Cham. https://doi.org/10.1007/978-3-031-16440-8_3
- J.L. Rumberger, E. Baumann, P. Hirsch, A. Janowczyk, I. Zlobec and D. Kainmueller (2022). Panoptic segmentation with highly imbalanced semantic labels. 2022 IEEE International Symposium on Biomedical Imaging Challenges (ISBIC), p. 1-4. https://doi.org/10.1109/ISBIC56247.2022.9854551
- P. Hirsch, L. Epstein, and L. Guignard (2020). Chapter 20 - Mathematical and bioinformatic tools for cell tracking. In: M. Schnoor, L-M. Yin, S.X. Sun (eds) Cell Movement in Health and Disease, Academic Press, 2022, p. 341-361, ISBN 9780323901956. https://doi.org/10.1016/B978-0-323-90195-6.00013-9
- C. Malin-Mayor, P. Hirsch, L. Guignard, K. McDole, Y. Wan, W.C. Lemon, D. Kainmueller, P.J. Keller, S. Preibisch, and J. Funke (2023). Automated reconstruction of whole-embryo cell lineages by learning from sparse annotations. Nat Biotechnol 41, 44–49. https://doi.org/10.1038/s41587-022-01427-7
Conference presentations
- P. Hirsch and D. Kainmueller. An Auxiliary Loss for Learning Nuclei Segmentation in 3D Microscopy Images. (Poster presentation), Frontiers in Imaging Science II, Janelia Research Campus, 1-4 May 2019.
- P. Hirsch, J.L. Rumberger, X. Yu, M. Dohmen, V.E. Guarino, A. Mokarian, L. Mais, J. Funke, and D. Kainmueller. What can go wrong with tile&stitch? (Poster presentation), Crick Bioimage Analysis Symposiym, London, U.K., 22-23 November 2021.
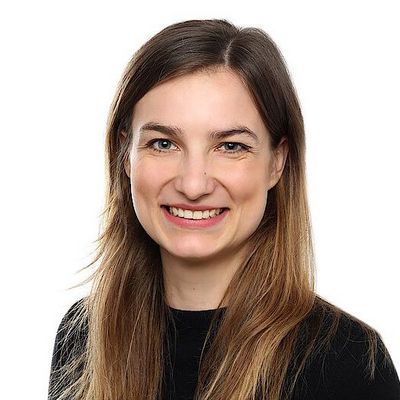
Alexandra Kapp
TU Berlin - HTW
Contact
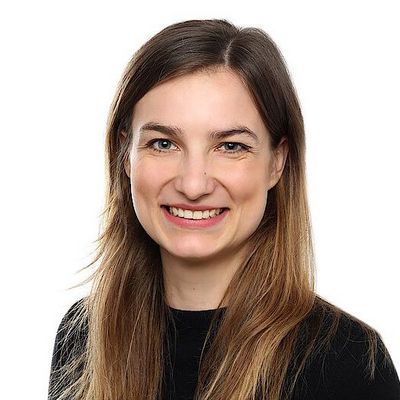
Associated Doctoral Researcher
Supervisors:
Human mobility data is a crucial resource for urban mobility applications, such as city planning, traffic modeling, routing applications, or mobility services. Mobility data can bring valuable benefits, but it does not come without personal reference. The implementation of measures such as anonymization is thus needed to protect individuals' privacy. Naturally, a trade-off between privacy and utility arises as such techniques decrease the data’s utility which potentially limits its use.
This work aims to identify, explore implement and evaluate privacy-preserving techniques for mobility data and their impact on the usability in real-world use cases and datasets. Practitioners will likely only adopt such methods if these do not highly impair practical usage. Also, methods need to be made understandable and they need to be easy to implement by the users in practice. Even though large tech companies, such as Apple, Google, and Microsoft already make use of privacy methods with differential privacy guarantees, there is still a gap between state-of-the-art privacy methods and common practices within the majority of companies.
As the impact on applications’ utility stays unclear, practitioners hesitate to implement such methods. This calls for a set of comprehensible utility metrics that quantify the impact on the utility and make different methods easily comparable. Also, academic research often lacks usable implementations for its theoretical solutions that allow easy reuse of the proposed methods. Lacking resources are therefore another hurdle, as the implementation of complex privacy-preserving methods needs time and expertise.
With this work, I want to contribute to the practical applicability of suitable privacy methods for human mobility data according to state-of-the-art privacy research.
Full-length publications
- A. Kapp (2022). Collection, usage and privacy of mobility data in the enterprise and public administrations. Proceedings on Privacy Enhancing Technologies. DOI 10.2478/popets-2022-0117
- A. Kapp, S. Nuñez von Voigt, H. Mihaljević, and F. Tschorsch (2022). Towards mobility reports with user-level privacy. Journal of Location Based Services, 21 Nov 2022. DOI 10.1080/17489725.2022.2148008
- A. Kapp, J. Hansemeyer, and H. Mihaljević (2023). Generative Models for Synthetic Urban Mobility Data: A Systematic Literature Review. ACM Computing Surveys. https://doi.org/10.1145/3610224
- A. Kapp, and H. Mihaljevic (2023). Reconsidering utility: unveiling the limitations of synthetic mobility data generation algorithms in real-life scenarios. In Proceedings of the 31st ACM International Conference on Advances in Geographic Information Systems (SIGSPATIAL '23), 93, 1–12. https://doi.org/10.1145/3589132.3625661
- A. Kapp, E. Hoffmann, E. Weigmann, and H. Mihaljević (2024). SurfaceAI: Automated creation of cohesive road surface quality datasets based on open street-level imagery. In Proceedings of the 2nd ACM SIGSPATIAL International Workshop on Advances in Urban-AI. doi: 10.1145/3681780.36972
Conference presentations
-
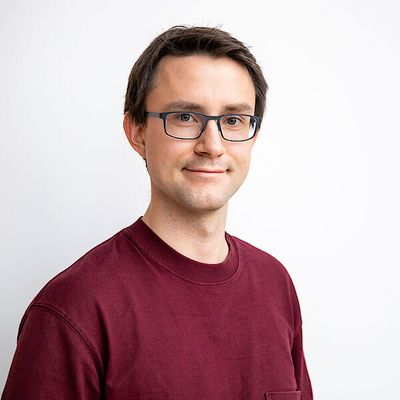
Thorren Kirschbaum (né Gimm)
HZB - FU Berlin
Contact
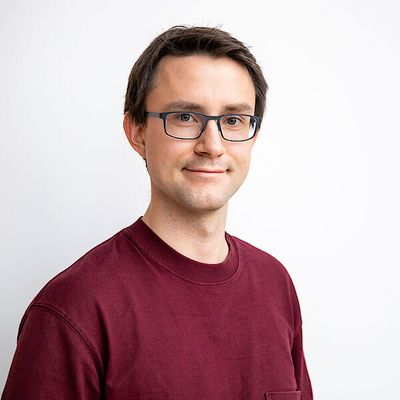
Supervisors:
The world is facing an ever-increasing demand for energy and resources as the scarcity of resources and the pollution of the environment are forcing us to redesign the foundations of global economies. New methods of producing „green“ energy and chemical base materials are in heavy demand. Hydrogen generated from environmentally neutral processes has the potential to provide both: a zero-emission energy carrier and chemical feedstock. However, the processes needed for the „clean“ production of hydrogen are not yet economically viable on a large scale. This project explores a novel way to generate hydrogen by splitting water into its elements, H2 and O2.
A key goal of modern energy research is to find efficient ways to achieve this splitting. The process relies on the efficient reduction of water hydrogen and oxidation of water oxygen. It has long been known that electrons solvated in water are the ideal, most direct agents to induce this reduction, but typically generating them has required harsh reaction conditions that have limited this approach. Very recently, however, a relatively mild production process was experimentally achieved using hydrogen-covered nanodiamonds illuminated by light. The process is conceived as follows: (1) The nanodiamond is excited and an electron moves towards the particle‘s surface, which permits (2) the electron to transfer into the interfacial water and (3) to move into the solution, where (4) it eventually reacts. Still we are far from understanding the precise mechanism underlying this effect, which is a key to improving and scaling-up its performance.
To learn more about the electron generating processes, we plan to model the electron transfer and solvation dynamics (described in (1)-(3) above) using coupled multi-scale electron and nuclear dynamics methods. Additionally, we will optimize the reaction paramters through a combination of quantum chemistry and machine learning. Steps (1-2) require intricate quantum electron dynamics (ED) calculations, which can be done only for a small number of molecular conformations. Steps (2-3) rely on electron hopping/transfer rates in conjunction with statistical interface physics and simulations of the molecular dynamics (MD) of the diamond/water interface. Deep learning will be used to approximate results from ED to parametrize MD simulations and create a time-dependent multi-physics description of the full process. This should give us a significantly better understanding of the system. Subsequently, we will use methods of optimal control to find the most efficient electron solvation process, in which the optimal control parameters are surface decoration, UV pulse (intensity, duration, shape), and temperature. Furthermore, the nanodiamonds‘ electronic properties will be optimized for excitation by sunlight through an approach that combines density functional theory (DFT) and supervised machine learning.
Doctoral thesis
T. Kirschbaum (2023). On the Electronic Structure of Nanodiamonds for Photocatalysis. Freie Universität Berlin.
Full-length publications
- J. Ren, L. Lin, K. Lieutenant, C. Schulz, D. Wong, T. Gimm, A. Bande, X. Wang, and T. Petit (2020). Role of dopants on the local electronic structure of polymeric carbon nitride photocatalysts. Small Methods 2000707. https://doi.org/10.1002/smtd.202000707
- T. Kirschbaum, T. Petit, J. Dzubiella, and A. Bande (2022). Effects of oxidative adsorbates and cluster formation on the electronic structure of nanodiamonds. J. Comput. Chem., 43,13, 923-929. https://doi.org/10.1002/jcc.26849
- F. Buchner, T. Kirschbaum, A. Venerosy, H. Girard, J-C. Arnault, B. Kiendl, A. Krueger, K. Larsson, A. Bande, T. Petit, and C. Merschjann (2022). Early dynamics of the emission of solvated electrons from nanodiamonds in water. Nanoscale, 14,17188-17195. https://doi.org/10.1039/D2NR03919B
- K. Palczynski, T. Kirschbaum, A. Bande, and J. Dzubiella (2023). Hydration Structure of Diamondoids from Reactive Force Fields. J. Phys. Chem. C, 127, 6, 3217–3227. https://doi.org/10.1021/acs.jpcc.2c07777
- T. Kirschbaum, B. von Seggern, J. Dzubiella, A. Bande, and F. Noé (2023). Machine Learning Frontier Orbital Energies of Nanodiamonds. J. Chem. Theory Comput. 19, 14, 4461–4473. https://doi.org/10.1021/acs.jctc.2c01275
- T. Kirschbaum, X. Wang, and A. Bande (2023). Ground and excited state charge transfer at aqueous nanodiamonds. J. Comput. Chem. https://doi.org/10.1002/jcc.27279
- X. Wang, P. Krause, T. Kirschbaum, K. Palczynski, J. Dzubiella and A. Bande (2024). Photo-excited charge transfer from adamantane to electronic bound states in water. Phys. Chem. Chem. Phys. https://doi.org/10.1039/D3CP04602H
Conference presentations
- T. Gimm, X. Wang, K. Palczynski, A. Bande, and J. Dzubiella. Nanodiamond-adsorbate interactions studied by DFT. (Poster presentation), Bunsen-Tagung 2021 - Multi-scale modelling & physical chemistry of colloids, Online, 10-12 May 2021.
- T. Gimm, X. Wang, K. Palczynski, A. Bande, and J. Dzubiella. Nanodiamond-adsorbate interactions studied by DFT. (Poster presentation), 57th Symposium of Theoretical Chemistry, Online, 20-24 September 2021.
- T. Kirschbaum, B. von Seggern, J. Dzubiella, A. Bande and F. Noé. Machine Learning Frontier Orbital Energies of Nanodiamonds. (Poster presentation), 58th Symposium of Theoretical Chemistry, Heidelberg, Germany, 18-22 September 2022.
- T. Kirschbaum, B. von Seggern, J. Dzubiella, A. Bande, and F. Noé. Machine Learning Frontier Orbital Energies of Nanodiamonds. (Oral presentation), Asia Pacific Conference of Theoretical and Computational Chemistry, Quy Nhon, Vietnam, 19-23 February 2023.
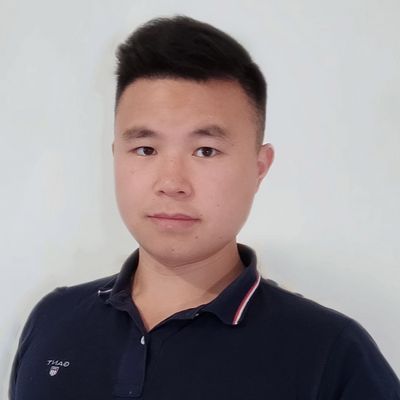
Rui Li
MDC - HZDR - TU Dresden
Contact
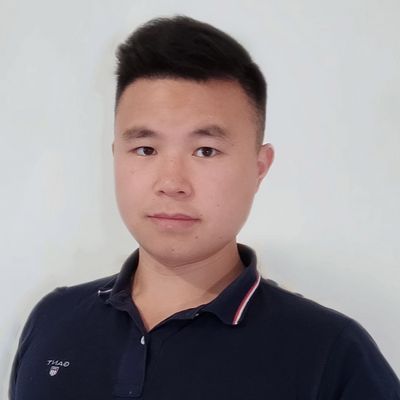
Supervisors:
Artur Yakimovich (HZDR, Dresden)
Ivo F. Sbalzarini (TU Dresden)
3D structure information of biological entities has a strong impact on drug screening and clinical experiments. Microscopy serves as a reliable tool for imaging the 3D structures - both electron microscopy (EM) and light microscopy (LM). On a nanoscale in EM, the Cryo-ET is advancing as a method to determine the biological structure within the entities’ native environment. However, higher time consumption and constraints on electron dose limit the potential of Cryo-ET. On a macro scale in LM, confocal fluorescence microscopy (CFM) obtains axial optical sections by filtering out out-focus light using a pinhole or a slit in the optical path of the microscope. This allows stacking the thin slices into a 3D volume. Yet, CFM comes with drawbacks such as high equipment costs and higher skill requirements in microscopy. In contrast, widefield microscopy is simple and ubiquitous in biomedical laboratories.
Machine learning (ML) serves as a promising end-to-end solution. For 3D model reconstruction in CV, the ML solutions show advantages by restoring 3D information based on limited 2D input images (e.g., single-images and multi-images). In the biology domain, scholars proposed the potential to enhance 3D microscopy performance with ML technology at an early stage. However, since then only few contributions have been made to 3D biological model reconstruction with newly composed ML theories (e.g., GAN, VAE, etc.).
In this work, we will focus on the 3D reconstructions from the focal series of LM and EM using deep neural networks (DNNs). Specifically in Cryo-EM, through electron-optical defocusing we could obtain 3D information of given molecules on the 2D focal planes. We hypothesize it is possible to restore 3D information of pleomorphic objects from 2D images. For LM, instead of the expensive and skills-taxing CFM, we will adopt the images from cheap widefield microscopes. By filtering out the out-focus pixels of images in focal planes through DNNs, We will explore the possibilities to recover 3D information from out-of-focus planes of non-confocal microscopic 3D stacks.
Full-length publications
- R. Li, V. Sharma, S. Thankgamani, and A. Yakimovich (2022). Open-Source Biomedical Image Analysis Models: A Meta-Analysis and Continuous Survey. Frontiers in Bioinformatics. https://doi.org/10.3389/fbinf.2022.912809
- R. Li, M. Kudryashev, and A. Yakimovich (2023). A weak-labelling and deep learning approach for in-focus object segmentation in 3D widefield microscopy. Sci Rep 13, 12275. https://doi.org/10.1038/s41598-023-38490-2
- R. Li, G. della Maggiora, V. Andriasyan, A. Petkidis, A. Yushkevich, M. Kudryashev, and A. Yakimovich (2024). Microscopy image reconstruction with physics-informed denoising diffusion probabilistic model. Nature Communication Engineering.
- R. Li, M. Kudryashev, and A. Yakimovich (2025). Solving the inverse problem of microscopy deconvolution with a residual Beylkin-Coifman-Rokhlin neural network. In: Computer Vision – ECCV 2024. doi.org/10.1007/978-3-031-73226-3_22
Conference presentations
- L. Rui, M. Kudryashev, and A. Yakimovich. Translate widefield microscopy images into the 3D models in confocal microscope style using deep neural networks. 6th International Symposium on Image-based Systems Biology (ibSB), Online & Jena, Germany, 8-9 September 2022.
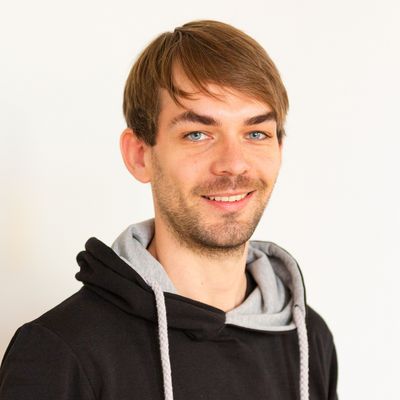
Henning Lilienkamp
GFZ - TU Berlin
Contact
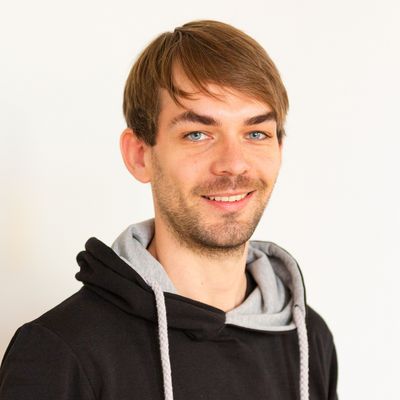
Doctoral thesis
H. Lilienkamp (2024). Enhanced computational approaches for data-driven characterization of earthquake ground motion and rapid earthquake impact assessment. University of Potsdam.
Supervisors:
In many regions of the world earthquakes pose a persistent threat to the built environment, especially with respect to the civil infrastructures that are now fundamental to our society. In the aftermath of recent earthquakes, such as the 2010‐2011 Christchurch (New Zealand) events, damage to road, railway and utility/communications networks may be the dominant contributor to economic loss, with socio‐economic impacts that can last for a long period after the event and impede the recovery. The importance of analysing the seismic risk and vulnerability of spatially distributed infrastructure networks is becoming widely recognized by engineers, insurers and the scientific community at large. Such analyses present a challenge to scientists and engineers due to the complex interactions between interconnected elements within the infrastructure. The statistical models require a computational complexity so large as to prohibit the real‐time assessment of the post‐event network state. Conversely, simplified models may fail to capture the correlations and dependencies within a system in its entirety. In this project we introduce novel machine learning techniques into this process to provide statistically robust assessments of the performance of a network, in terms of both connectivity and flow, that would allow for rapid evaluation of the impact of an event for use in the immediate aftermath and recovery phase, or as part of a probabilistic assessment of economic loss.
Full-length publications
- H. Lilienkamp, S. von Specht, G. Weatherill, G. Caire, and F. Cotton (2022). Ground-motion modeling as an image processing task: Introducing a neural network based, fully data-driven, and nonergodic approach. Bull. Seismol. Soc. Am. 112, 1565–1582. https://doi.org/10.1785/0120220008
- H. Lilienkamp, R. Bossu, F. Cotton, F. Finazzi, M. Landès, G. Weatherill, and S. von Specht (2023). Utilization of Crowdsourced Felt Reports to Distinguish High‐Impact from Low‐Impact Earthquakes Globally within Minutes of an Event. The Seismic Record, 3 (1): 29–36. https://doi.org/10.1785/0320220039
Conference presentations
- H. Lilienkamp, G. Weatherill, F. Cotton, and G. Caire. The role of spatial cross-correlation structures of ground motion fields forseismic risk assessment of spatially distributed assets and infrastructurenetworks. (Poster presentation), EGU General Assembly, Vienna, Austria, 7–12 April 2019.
- H. Lilienkamp, S. Specht, G. Weatherill, and F. Cotton. Exploring the physics and uncertainties in spatial cross-correlation models for ground motion intensity measures. (Oral presentation), AGU General Assembly, San Francisco, USA, 9–13 December 2019.
- H. Lilienkamp, F. Cotton, G. Caire, G. Weatherill, and S. von Specht. Fully data-driven, partially non-ergodic ground motion modeling using convolutional neural networks. (Poster presentation), Taiwan Earthquake Research Center Annual Meeting, Taiwan, 20-22 October 2020.
-
H. Lilienkamp, R. Bossu, F. Cotton, F. Finazzi, G. Weatherill, and S. von Spech. Utilization of crowdsourced macroseismic observations to distinguish “high-impact” from “low-impact” earthquakes globally within minutes of an event. (Oral presentation), IUGG23 General Assembly, Berlin, Germany, 11-20 July 2023.
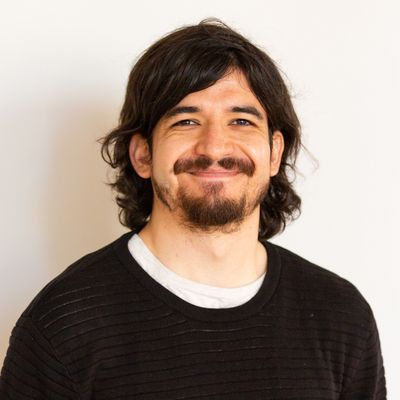
Nicolas Miranda
HU Berlin - DESY
Contact
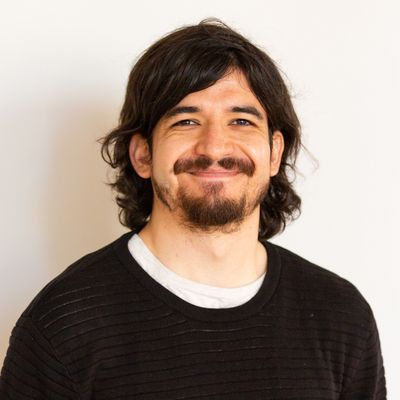
(Interrupted studies before PhD degree)
Supervisors:
The Universe holds several avenues for the (catastrophic) end of stars. These include their gravitational collapse to a Neutron star, resulting in a so-called core-collapse Supernova, stars being swallowed by the central Black Hole of a galaxy, as well as Kilonova, the result of two merging Neutron stars recently detected for the first through the electromagnetic follow-up of a Gravitational wave event. The diversity of energetic and explosive events serves as a laboratory for fundamental physics that is explored through increasingly powerful observational facilities. With the start of the Zwicky Transient Facility (ZTF), the detection rate of time-variable phenomena in the Universe will increase by a factor 10 compared to existing surveys, far beyond what can be manually examined by astronomers. This PhD project focuses on developing new data management and machine learning approaches that will allow the scalable analysis of ZTF data through the implementation of flexible/scalable data infrastructure for classifying new transients. As the computing resources needed for this kind of computation will vary, there is also the need to manage them in an elastic manner thus leading to new monitoring and resource management strategies.
Full-length publications
- J. Nordin, V. Brinnel, J. van Santen, ..., M. Kowalski, A. Mahabal, N. Miranda, ..., and C. Ward (2019). Transient processing and analysis using AMPEL: Alert management, photometry and evaluation of light curves. Astronomy & Astrophysics, 631, A147. https://doi.org/10.1051/0004-6361/201935634
- Y. Yao, S.R. Kulkarni, K.B. Burdge, …, N. Miranda, ..., and M.T. Soumagnac (2021). Multi-wavelength observations of AT2019wey: A new candidate black hole low-mass X-ray binary. The Astrophysical Journal, 920(2), 120. https://doi.org/10.3847/1538-4357/ac15f9
- N. Miranda, J-C. Freytag, J. Nordin, R. Biswas, V. Brinnel, C. Fremling, ..., and J. van Santen (2022). SNGuess: A method for the selection of young extragalactic transients. Astronomy & Astrophysics, 665, A99. https://doi.org/10.1051/0004-6361/202243668
Conference presentations
-
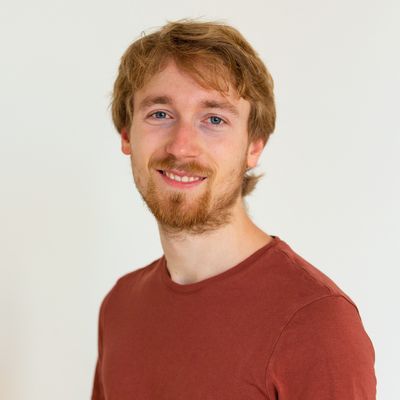
Jannes Münchmeyer
GFZ - HU Berlin
Contact
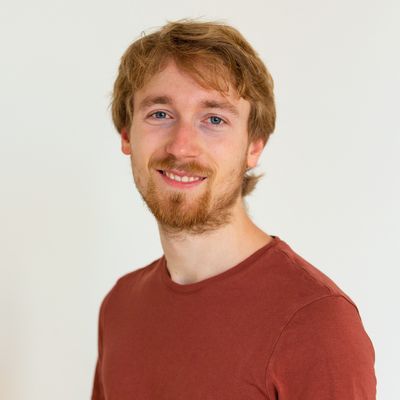
Supervisors:
Earthquakes count among the largest natural threats to humans. The current state of research suggests that it will not be possible to predict earthquakes reliably anytime in the near future. What is possible, on the other hand, is to provide reliable early warning in the context of ongoing earthquakes. The goal is to provide warnings a few seconds before strong shaking occurs. Such warnings can trigger automatic reactions, like stopping trains, or alert humans early enough to allow them to seek cover.
Usually, early warnings are based on recording the early, relatively weak shaking caused by an earthquake, inferring its size, and then predicting the level of shaking to follow. However, a look at the physics behind earthquakes reveals a crucial issue in attempting to make such predictions. An earthquake emits seismic waves from a rapidly growing rupture between two tectonic plates. These ruptures can traverse distances of tens or even hundreds of kilometers, and consequently, even when a rupture grows quickly, it might take tens of seconds or even minutes for the full rupture to occur.
There is no scientific consensus on how accurately the size of an earthquake can be assessed at what time during an ongoing rupture. There are two basic positions among experts: one holds that the size of an earthquake can be accurately predicted from its onset or during the first few seconds, and the other that accurate assessment is impossible until the rupture is largely finished. Which of these positions is correct will have a profound impact on the potential of early warning methods: if the earthquake's size can only be determined at the end of the rupture, then only short warning times – if any at all – will be possible.
In this PhD project, I am taking a novel, data-driven approach to the question of predictability. Using machine learning, I will build real-time assessment systems to predict the size of an event during an ongoing rupture. If we can design a model that can accurately assess the size of an earthquake from its first seconds, this will be a demonstration that ruptures can be feasibly predicted. A further step will be to integrate our real-time assessment model into earthquake early warning systems, to improve their performance with our state-of-the-art estimation methodology.
Doctoral thesis
J. Münchmeyer (2022). Machine learning for fast and accurate assessment of earthquake source parameters. Humboldt Universität Berlin. doi: 10.18452/25174
Full-length publications
- L. Weber, J. Münchmeyer, T. Rocktäschel, M. Habibi, and U. Leser (2019). HUNER: Improving biomedical NER with pretraining. Bioinformatics, 36(1), 295-302. 10.1093/bioinformatics/btz528
-
L. Weber, P. Minervini, J. Münchmeyer, U. Leser, and T. Rocktäschel (2019). NLProlog: Reasoning with weak unification for question answering in natural language. In Proceedings of the 57th Annual Meeting of the Association for Computational Linguistics, 6151-6161. 10.18653/v1/P19-1618
-
J. Münchmeyer, D. Bindi, C. Sippl, U. Leser, and F. Tilmann (2019). Low uncertainty multi-feature magnitude estimation with 3D corrections and boosting tree regression: Application to North Chile. Geophysical Journal International, 220(1), 142-159. doi.org/10.1093/gji/ggz416
-
J. Münchmeyer, D. Bindi, U. Leser, and F. Tilmann (2020). The transformer earthquake alerting model: A new versatile approach to earthquake early warning. Geophysical Journal International, ggaa609. doi.org/10.1093/gji/ggaa609
-
L. Weber, M. Sänger, J. Münchmeyer, M. Habibi, U. Leser, and A. Akbik (2021). HunFlair: An easy-to-use tool for state-of-the-art biomedical Named Entity Recognition. Bioinformatics, btab042, https://doi.org/10.1093/bioinformatics/btab042
-
J. Münchmeyer, D. Bindi, U. Leser, and F. Tilmann (2021). Earthquake magnitude and location estimation from real time seismic waveforms with a Transformer Network. Geophysical Journal International, 226(2), 1086-1104. https://doi.org/10.1093/gji/ggab139
-
W.J. Foster, G. Ayzel, J. Münchmeyer, T. Rettelbach, N. Kitzmann, T.T. Isson, M. Mutti, and M. Aberhan (2021). Machine learning identifies ecological selectivity patterns across the end-Permian mass extinction. Paleobiology, 1-15. https://doi.org/10.1017/pab.2022.1
-
L. Weber, S. Garda, J. Münchmeyer, and U. Leser (2021). Extend, don’t rebuild: Phrasing conditional graph modification as autoregressive sequence labelling. In Proceedings of the 2021 Conference on Empirical Methods in Natural Language Processing, 1213–1224.
-
J.* Münchmeyer, J.* Woollam, F. Tilmann, A. Rietbrock, D. Lange, ..., and H. Soto (2021). Which picker fits my data? A quantitative evaluation of deep learning based seismic pickers. Journal of Geophysical Research: Solid Earth, 127, 1, e2021JB023499. https://doi.org/10.1029/2021JB023499 *Equal contribution
-
K. Singh, J. Münchmeyer, L. Weber, U. Leser and A. Bande (2022). Graph Neural Networks for Learning Molecular Excitation Spectra. J. Chem. Theory Comp., 18, 7, 4408-4417. DOI: 10.1021/acs.jctc.2c00255
-
J.* Woollam, J.* Münchmeyer, F. Tilmann, A. Rietbrock, D. Lange, ..., and H. Soto (2022). SeisBench - A Toolbox for Machine Learning in Seismology. Seismological Research Letters, 93(3), 1695–1709. https://doi.org/10.1785/0220210324 *Equal contribution
-
J. Münchmeyer, U. Lesera and F. Tilmann (2022). A probabilistic view on rupture predictability: All earthquakes evolve similarly. Geophysical Research Letters, 49, 13, e2022GL098344. https://doi.org/10.1029/2022GL098344
Conference presentations
- J. Münchmeyer, D. Bindi, C. Sippl, and F. Tilmann. Increasing magnitude scale consistency by combining multiple waveform features through machine learning. (Oral presentation), EGU General Assembly, Vienna, 7-12 April 2019.
- J. Münchmeyer, D. Bindi, U. Leser, and F. Tilmann. Convolutional event embeddings for fast probabilistic earthquake assessment. (Poster presentation), AGU Fall Meeting, San Francisco, USA, 9-13 December 2019.
- J. Münchmeyer, D. Bindi, U. Leser, and F. Tilmann. End-to-end PGA estimation for earthquake early warning using transformer networks. (Oral presentation), EGU General Assembly, Online, 4-8 May 2020.
- J. Münchmeyer, D. Bindi, U. Leser, and F. Tilmann. The Transformer Earthquake Alerting Model: Improving Earthquake Early Warning with Deep Learning. (Oral presentation), AGU Fall Meeting, Online, 13-17 December 2020.
- J. Münchmeyer, D. Bindi, U. Leser, and F. Tilmann. Insights into deep learning for earthquake magnitude and location estimation. (PICO presentation), EGU General Assembly, Online, 19-30 April 2021. https://doi.org/10.5194/egusphere-egu21-4718
- J. Münchmeyer, D. Bindi, U. Leser, and F. Tilmann. The Transformer Earthquake Alerting Model: A Data Driven Approach to Early Warning. (Oral presentation), Seismological Society of America (SSA) Annual Meeting, Online, 19-23 April 2021.
- J. Münchmeyer, J. Woollam, ..., D. Lange, A. Rietbrock, and F. Tilmann. SeisBench: A framework for machine learning in seismology. (Oral presentation), 37th General Assembly of the European Seismological Commission, Online, 19-24 September 2021.
- J. Münchmeyer, U. Leser, and F. Tilmann. A probabilistic view of earthquake rupture predictability. (Oral presentation), AGU Fall Meeting, Online & New Orleans, USA, 13-17 December 2021.
- J. Münchmeyer, J. Woollam, F. Tilmann, A. Rietbrock, D. Lange, ..., and H. Soto. Which picker fits my data? A quantitative evaluation of deep learning based seismic pickers. EGU General Assembly 2022, Vienna, Austria, 23–27 May 2022. https://meetingorganizer.copernicus.org/EGU22/EGU22-4071.html
- J. Münchmeyer, J. Woollam, F. Tilmann, A. Rietbrock, D. Lange, ..., and H. Soto. (2022). SeisBench: A toolbox for machine learning in seismology. Helmholtz AI Conference, Dresden, Germany, 2-3 June 2022.
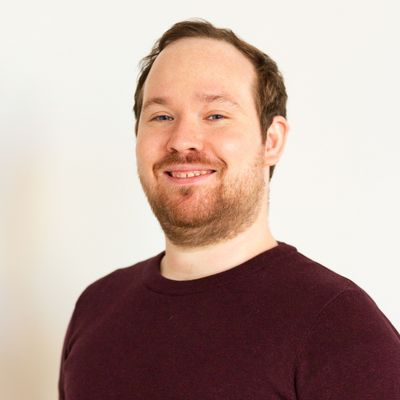
Gregor Pfalz
AWI - HU Berlin
Contact
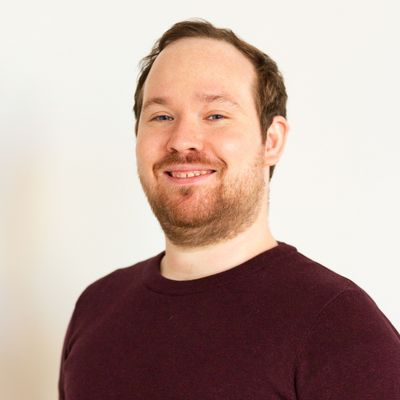
Supervisors:
The goal of this PhD is to detect the past ecosystem‐climate relationships in Arctic lake settings by big data analytics of a polar proxy dataset. We focus on two topics: Data management and data science ‐ development of a data analytics system for palaeolimnological proxy data designed for multivariate statistics. Geoscience ‐ Past and present environmental dynamics in Arctic landscapes and their impact on polar lake ecosystems. A unique, standardized, data set of proxy data from lake sediment cores in the Eastern Arctic will be compiled using the new PALIM Database. To correlate ecosystem changes with climate changes, multivariate statistics will be performed on quality controlled biotic and abiotic proxy data. The objective of this project is to develop a state‐of‐the‐art data analytics system that allows to detect the main relationships of ecosystem dynamics and climate changes and their spatiotemporal pattern in dependence to lake attributes, i.e. thermokarst or glacial origin, landscape‐type, lake‐ecosystem‐type, lake age, and catchment‐vegetation.
Doctoral thesis
G. Pfalz (2024). Advancing knowledge of Arctic lake system dynamics: A data-driven perspective on spatiotemporal patterns. University of Potsdam. https://doi.org/10.25932/publishup-63655
Full-length publications
- H. Grotheer, V. Meyer, T. Riedel, G. Pfalz, L. Mathieu, J. Hefter, ..., and M. Fritz (2020). Burial and origin of permafrost‐derived carbon in the nearshore zone of the southern Canadian Beaufort Sea. Geophysical Research Letters, 47, e2019GL085897. https://doi.org/10.1029/2019GL085897
- G. Pfalz, B. Diekmann, J-C. Freytag, and B.K. Biskaborn (2021). Harmonizing heterogeneous multi-proxy data from lake systems. Computers & Geosciences. https://doi.org/10.1016/j.cageo.2021.104791
- B.K. Biskaborn, L. Nazarova, T. Kröger, L.A. Pestryakova, L. Syrykh, G. Pfalz, U. Herzschuh, and B. Diekmann (2021). Late quaternary climate reconstruction and lead-lag relationships of biotic and sediment-geochemical indicators at lake Bolshoe Toko, Siberia. Front. Earth Sci., 9, 703. https://doi.org/10.3389/feart.2021.737353
- S.A. Vyse, U. Herzschuh, G. Pfalz, L.A. Pestryakova, B. Diekmann, N. Nowaczyk, and B.K. Biskaborn (2021). Sediment and carbon accumulation in a glacial lake in Chukotka (Arctic Siberia) during the late Pleistocene and Holocene: Combining hydroacoustic profiling and down-core analyses. Biogeosciences. https://doi.org/10.5194/bg-18-4791-2021
- L. Hughes-Allen, F. Bouchard, C. Hatté, H. Meyer, L.A. Pestryakova, G. Pfalz, B. Diekmann, D.A. Subetto, and B.K. Biskaborn (2021). 14 000-year carbon accumulation dynamics in a Siberian lake reveal catchment and lake productivity changes. Front. Earth Sci., 9, 1–19. https://doi.org/10.3389/feart.2021.710257
- G. Pfalz, B. Diekmann, J-C. Freytag, L. Sryrkh, D.A. Subetto, and B.K. Biskaborn (2022). Improving age-depth correlations by using the LANDO model ensemble. Geochronology, 4, 269–295. https://doi.org/10.5194/gchron-4-269-2022
- G. Pfalz, B. Diekmann, J-C. Freytag, and B.K. Biskaborn (2023). Effect of temperature on carbon accumulation in northern lake systems over the past 21,000 years. Front. Earth Sci., Sec. Quaternary Science, Geomorphology and Paleoenvironment, 11. https://doi.org/10.3389/feart.2023.1233713
Conference presentations
- G. Pfalz, B. Diekmann, J-C. Freytag, and B.K. Biskaborn. Decipher Arctic lakes ecosystem dynamics. (Poster presentation), YES Congress, Berlin, Germany, September 9-13, 2019.
- G. Pfalz, B. Diekmann, J-C. Freytag, and B.K. Biskaborn. Harmonizing heterogeneous multi-proxy data from Arctic lake sediment records. (PICO presentation), EGU General Assembly, Online, 19–30 April 2021, EGU21-9401. https://doi.org/10.5194/egusphere-egu21-9401
- G. Pfalz, B. Diekmann, J-C. Freytag, L. Syrykh, D.A. Subetto, and B.K. Biskaborn, Using LANDO as a universal wrapper for applying multiple age-depth modeling systems for sediment records from Arctic lake systems. EGU General Assembly 2022, Vienna, Austria, 23–27 May 2022. https://doi.org/10.5194/egusphere-egu22-8743
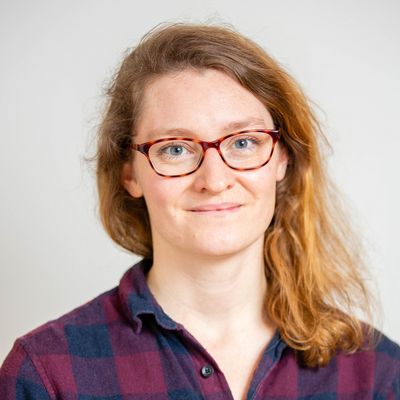
Tabea Rettelbach
AWI - HU Berlin
Contact
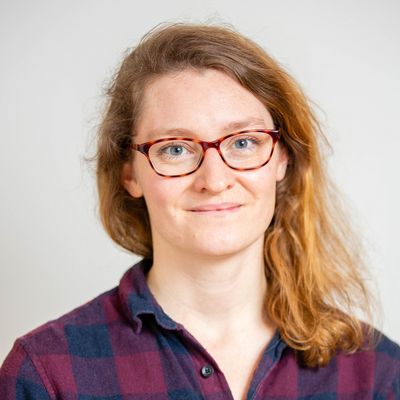
Supervisors:
Guido Grosse (AWI)
Johann-Christoph Freytag (HUB)
Temperatures in the Arctic are warming. They’re warming even more rapidly than in other regions of the World. Unfortunately, due to their remoteness, these highly sensitive environments are not fully researched and explored yet, and thus represent a significant unknown in our Earth system models and our general understanding of their importance.
One of the major components of the Arctic is permafrost, which describes any ground that stays at, or below 0 °C for at least two consecutive years. Permafrost covers around one fourth of the landmass in the northern hemisphere and can reach depths of up to 1000 meters. With the warming atmosphere however, these soils are recently also experiencing higher temperatures, and large regions are starting to thaw. In the thawing ground, bacteria are now able to decompose century-old organic carbon, effectively releasing large amounts of carbon dioxide into the atmosphere, thus amplifying the atmospheric greenhouse effect even further. Researchers estimate that this permafrost holds up to 1400 Gt of carbon dioxide, about twice the amount that is currently in our planet’s atmosphere. It is therefore crucial for us to understand the rate of permafrost degradation in order to make the most accurate predictions for our climate and estimate its significance to life on Earth.
One of multiple possibilities for monitoring permafrost regions, is via remote sensing, where we can make use of aerial imagery (i.e., from satellites, airplanes, drones) to observe and analyze features at the ground’s surface that are very specific for permafrost soils. One example of such characteristic landscapes is polygonal thermokarst. These landscapes are characterized by large polygons (approximately 10-50 m across) that, in the undegraded state, show elevated rims at their borders. With an ongoing warming, these rims will however degrade and erode to become lower troughs between these polygons. With ongoing degradation, single troughs will gradually connect and the network of channels between the polygons steadily grows. A higher connectivity of troughs thus advances the drainage of surface water for entire landscapes. By evaluating digital elevation models in combination with multispectral imagery (in the RGB and near-infrared wavelengths), we can observe this process of degradation and permafrost thaw as well as the hydrological state of the landscape from above.
So far, studies on quantifying this trough connectivity are limited to local field studies and regional hydrological models only. My research therefore focuses on the extraction of trough characteristics from high spatial resolution aerial imagery and digital elevation data, to model this network of channels as a graph (a concept for network analysis from discrete mathematics and computer sciences). Graph theory provides a multitude of metrics that allow the analysis of underlying network characteristics such as the connectivity of single channels, the intensity of the connections, but also width and depth of single troughs (which can be stored as edge weights). The figure below shows some preliminary results of the terrain analysis of a study area in northern Alaska, USA. Further, by evaluating the graphs of channels from multiple steps in time, it is possible to analyze the rate of degradation. This can be inferred when the merging of multiple smaller graphs into fewer larger graphs occurs, when certain connections arise, but also from the increasing values of depth and width of the channels as time advances. With sufficiently available data and dense time series, even the prediction of future network development is possible.
Being able to monitor the hydrological regime of a landscape and quantifying the local thaw rate of permafrost is crucial in order to gain insight into its contribution to greenhouse gases in the atmosphere and therefore its role in climate change. On a methodological level, processing this type of information as graphs, opens up possibilities to consider even small-scale changes on a trough-by-trough level and offers significantly shorter computing times as compared to calculations based on spatial raster-imagery. This allows analysis coverage of much larger regions with available processing capacities, which is an important step towards a pan-Arctic and holistic understanding of the Earth’s permafrost.
Full-length publications
- T. Rettelbach, M. Langer, I. Nitze, B. Jones. V. Helm, J-C. Freytag, and G. Grosse (2021). A quantitative graph-based approach to monitoring ice-wedge trough dynamics in polygonal permafrost landscapes. Remote Sens. 13, 3098. https://doi.org/10.3390/rs13163098
- W.J. Foster, G. Ayzel, J. Münchmeyer, T. Rettelbach, N. Kitzmann, T.T. Isson, M. Mutti, and M. Aberhan (2021). Machine learning identifies ecological selectivity patterns across the end-Permian mass extinction. Paleobiology, 1-15. https://doi.org/10.1017/pab.2022.1
- T. Rettelbach, M. Langer, I. Nitze, B. Jones, V. Helm, J-C. Freytag, and G. Grosse (2022). From images to hydrologic networks - Understanding the Arctic landscape with graphs. In ACM Proceedings of the 34th International Conference on Scientific and Statistical Database Management (SSDBM 2022). https://doi.org/10.1145/3538712.3538740
- N.H. Chan, M. Langer, B. Juhls, T. Rettelbach, P. Overduin, K. Huppert and J. Braun (2023). An Arctic Delta Reduced Complexity Model and its Reproduction of Key Geomorphological Structures. Earth Surface Dynamics, 259–285. https://doi.org/10.5194/esurf-11-259-2023
- W.J. Foster, B. J. Allen, N.H. Kitzmann, J. Münchmeyer, T. Rettelbach, J.D. Witts, , R.J. Whittle, E. Larina, M.E. Clapham and A.M. Dunhill (2023). How predictable are mass extinction events? Royal Society Open Science, 10 (3), 221507. https://doi.org/10.1098/rsos.221507
- B.M. Jones, S. Schaeffer Tessier, T. Tessier, M. Brubaker, M. Brook, J. Schaeffer, M.K. Ward Jones, G. Grosse, I. Nitze, T. Rettelbach, S. Zavoico, J.A. Clark, and K.D. Tape (2023). Integrating local environmental observations and remote sensing to better understand the life cycle of a thermokarst lake in Arctic Alaska. Arctic, Antarctic, and Alpine Research, 55(1).
- T. Rettelbach, I. Nitze, I. Grünberg, J. Hammar, S. Schäffler, D. Hein, M. Gessner, T. Bucher, J. Brauchle, J. Hartmann, T. Sachs, J. Boike, and G. Grosse (2023). Aerial imagery datasets of permafrost landscapes in Alaska and northwestern Canada acquired by the Modular Aerial Camera System. PANGAEA. https://doi.pangaea.de/10.1594/PANGAEA.961577
- T. Rettelbach, I. Nitze, I. Grünberg, J. Hammar, S. Schäffler, D. Hein, M. Gessner, T. Bucher, J. Brauchle, J. Hartmann, T. Sachs, J. Boike, and G. Grosse (2023). Super-high-resolution aerial imagery datasets of permafrost landscapes in Alaska and northwestern Canada. Earth System Science Data Discussions. 1-35. https://doi.org/10.5194/essd-2023-193 [Preprint]
Conference presentations
- T. Rettelbach, M. Langer, I. Nitze, B. Jones. J. Boike, J-C. Freytag, and G. Grosse. Potential von Graphen für die quantitative Analyse von tauenden Eiskeilpolygonnetzwerken. (Oral presentation), 11. Treffen des AK Permafrost der DGP, Online, 11 December 2020.
- T. Rettelbach, G. Grosse, I. Nitze, J. Brauchle, T. Bucher, M. Gessner, B.M. Jones, J. Boike, M. Langer, and J-C. Freytag. A quantitative graph-based assessment of ice-wedge trough dynamics in polygonal thermokarst landscapes of the Anaktuvuk river fire scar. (Oral presentation), AGU Fall Meeting, Online, 1-17 December 2020.
- T. Rettelbach, M. Langer, I. Nitze, B. Jones, V. Helm, J-C. Freytag, and G. Grosse. Quantifying erosional dynamics in ice-wedge networks with computer vision and graph theory. (Oral presentation), Regional Conference on Permafrost, Online, 24-29 October 2021.
- T. Rettelbach, M. Langer, I. Nitze, B. Jones, V. Helm, J-C. Freytag, and G. Grosse. Evaluating the effects of tundra fires on soil microtopography and hydrologic surface networks in polygonal permafrost landscapes. (Oral presentation), AGU Fall Meeting, News Orleans / Online, USA, 13-17 December 2021.
- T. Rettelbach, I. Nitze, and G. Grosse. Polar-6 airborne expedition Perma-X West Alaska 2021. (Oral presentation), 12. Treffen des AK Permafrost der DGP, Online, 06 May 2022.
- T. Rettelbach, I. Nitze, S. Schäffler, S. Barth, I. Grünberg, J. Hammar, M. Gessner, T. Bucher, J. Brauchle, T. Sachs, J. Boike, and G. Grosse. Super-high-resolution Earth observation datasets of North American permafrost landscapes. (Oral and poster presentation), 8th NASA ABoVE Science Team Meeting, Fairbanks, Alaska, USA, 9-12 May 2022.
- T. Rettelbach, C. Witharana, A. Liljedahl, M. Langer, I. Nitze, J-C. Freytag, and G. Grosse. The evolution of ice-wedge polygon networks in tundra fire scars. (Poster presentation), 16th International Circumpolar Remote Sensing Symposium, Fairbanks, Alaska, USA, 16-20 May 2022.
- T. Rettelbach, M. Langer, I. Nitze, V. Helm, J-C. Freytag, and G. Grosse. Quantifying rapid permafrost thaw with computer vision and graph theory. (Poster presentation), ESA Living Planet Symposium, Bonn, 23-27 May 2022.
- T. Rettelbach, M. Langer, I. Nitze, B. Jones, V. Helm, J-C. Freytag, and G. Grosse. From images to hydrologic networks - Understanding the Arctic landscape with graphs. (Oral presentation), 34th International Conference on Scientific and Statistical Database Management, Copenhagen, Denmark, 6-8 July 2022.
- T. Rettelbach, K. Heidler, N. Lehmann, I. Nitze, M. Langer, X. Zhu, J-C. Freytag, G. Grosse, and D. Kainmüller. Cross-resolution image segmentation for mapping smallest ponds in the Arctic. (Poster presentation), AI4EO Symposium 2023, Munich, Germany, 9-10 October 2023.
- T. Rettelbach, K. Heidler, N. Lehmann, I. Nitze, M. Langer, X. Zhu, J-C.Freytag, G. Grosse, and D. Kainmüller. Cross-resolution image segmentation for mapping smallest ponds in the Arctic. (Poster presentation), Helmholtz AI Conference, Düsseldorf, Germany, 12-14 June 2024.
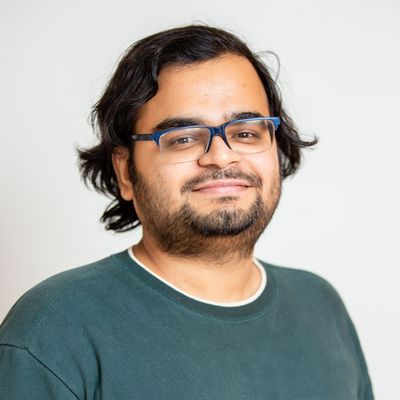
Kanishka Singh
HZB - HU Berlin
Contact
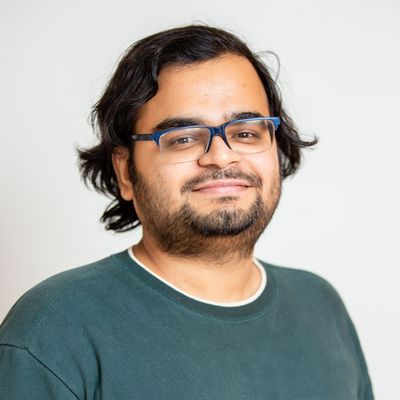
Supervisors:
In recent years data science more and more finds its way into the field of materials sciences, usually in the form of regression or classification approaches which are trained on known properties of certain materials and applied to predict these properties for less well characterized yet physically and chemically similar other materials. However, a pertinent problem in this field is the search for materials which are optimal with regard to some property, a problem which only partly matches to the above described scenario, since it is impossible to compute properties of the typically exponentially many candidate structures. For such a setting, the methods for calculating the properties of given structures must be complemented by intelligent algorithms which decide which structures are assessed in which order, and use the results of previous assessments to guide this search process through the space of all candidates. In this project, we research such methods and apply them to a particular important problem in the development of Solar Fuels: The search for an optimal material for photocatalysis, namely water oxidation or even water splitting. The searched material class will be sustainable grapheneoxide quantum dots. The desired properties are: (1) a band edge of more than 1.23 eV that is to be centered around the energies for the water-to-oxygen oxidation and the water-to-hydrogen reduction, (2) a good representation of its diverse spectra (X-ray absorption and emission (XAS and XES), UV/vis, and infrared), (3) sizes of the quantum dots, and (4) their oxygen and eventually dopand content. Many of these properties can be computed numerically with standard quantum chemistry software as e.g. the ORCA program, but only at the cost of extremely resource-intensive calculations which currently limits the number of materials that can be characterized. We study methods that allow a goal-driven search through the space of all candidate materials to find those that maximize a cost function derived from the materials properties. To this end, we plan to apply methods for reinforcement learning and heuristic search procedures, possibly combined with Deep Learning network to model correlations between the target properties and other, material-derived features. Particular challenges in this project are the inhomogeneity and incompleteness of data with respect to the target properties. The expected impact is high, because the project will create a complete workflow from high-throughput data production, database generation, and learning of structureproperty relationships that can as well be applied to many other interesting materials and classes of properties in the material sciences.
Doctoral thesis
K. Singh (2023). Machine Learning applications in spectroscopy and dynamics. Free University Berlin. http://dx.doi.org/10.17169/refubium-43143
Full-length publications
- K. Singh, J. Münchmeyer, L. Weber, U. Leser, and A. Bande (2022). Graph Neural Networks for Learning Molecular Excitation Spectra. J. Chem. Theory Comp., 18, 7, 4408-4417. DOI: 10.1021/acs.jctc.2c00255
- A. Kotobi, K. Singh, D. Höche, S. Bari, R. Meißner, and A. Bande (2023). Integrating Explainability into Graph Neural Network Models for the Prediction X-ray Absorption Spectra. J. Am. Chem. Soc., 145, 22584. https://doi.org/10.1021/jacs.3c07513
- K. Singh, K. H. Lee, D. Peláez, and A. Bande (2024). Accelerating wavepacket propagation with machine learning. J. Comput. Chem., 1. https://doi.org/10.1002/jcc.27443
Conference presentations
- K. Singh. Machine Learning for Quantum Dynamics. (Oral presentation), Asia Pacific Conference of Theoretical and Computational Chemistry, Quy Nhon, Vietnam, 19-23 February 2023.
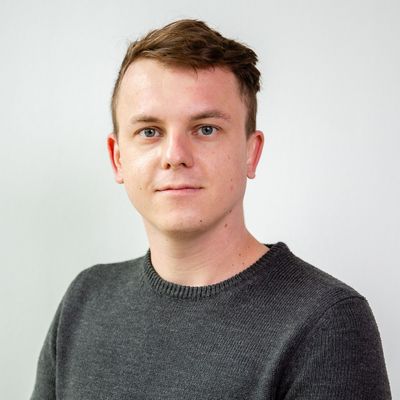
Kevin Styp-Rekowski
TU Berlin - GFZ
Contact
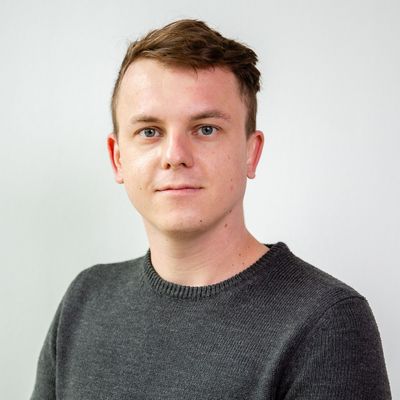
Supervisors:
Over the past few decades, high-precision magnetic satellite missions have been steadily providing new insights into the Earth’s magnetic field and the processes that underlie it. Yet we do not have a full picture of the geophysical mechanisms by which the geomagnetic field is created. Electric currents flowing along polar geomagnetic field lines are an important mechanism by which energy is transferred between the magnetosphere at several Earth radii and the ionosphere, the ionised part of the upper atmosphere, which lies at an altitude of 100-300 km altitude. These field-aligned currents (FAC) cannot be detected on ground, but they produce significant signatures in observations of the magnetic field made by Low-Earth-Orbiting satellites, sometimes even becoming visible as auroral lights. These currents are highly fluctuating and high-precision magnetic field missions such as CHAMP and Swarm have been used to characterize them.
An international consortium has started an initiative to make us of data from other sources. A number of satellites carry magnetometers that were not originally designed for scientific applications, but rather for the purposes of navigation – they include Cryosat, GOCE, GRACE-FO, and many others. A careful calibration of data from these magnetometers permits extractions of the signature of currents from the Earth's magnetic field. Combining such data from several satellites will provide an unprecedented level of global coverage and should strongly enhance our ability to particularly monitor the polar ionosphere and its interaction with the magnetosphere. This data and a higher level of coverage can play an important role in understanding short-lived magnetic storms, which have a large impact on a variety of domains.
To achieve this, we can draw on a global dataset that has been collected continuously from diverse satellite missions since the year 2000. Combining all of this data into a common mapping procedure is challenging: it comprises different sampling rates, signal amplitudes, noise levels, and latency. Combining data of different sampling rate, signal amplitude, noise level, and latency in one mapping procedure requires special care for data handling and inter-calibration to achieve an unbiased result uniformly valid over the globe. The amplitude of the signal detected is subject to variation and noise due to descending satellite orbits, the architectural settings of missions and naturally varying levels of solar flux. This means that particular attention must be paid to data handling and inter-calibration to achieve an unbiased result that is uniformly valid across the globe. Our initial results, based on data collected from for the GRACE-FO1 satellite, show that it is indeed possible to detect FACs by calibrating platform magnetometer data, as shown in the attached figures.
Doctoral thesis
K. Styp-Rekowski (2024). Machine Learning Calibration of Satellite Platform Magnetometer Data. University of Rostock.
Full-length publications
- K. Styp-Rekowski, C. Stolle, I. Michaelis, and O. Kao (2021). Calibration of the GRACE-FO satellite platform magnetometers and co-estimation of intrinsic time shift in data. IEEE International Conference on Big Data, 5283-5290. https://doi.org/10.1109/BigData52589.2021.9671977
-
C. Stolle, I. Michaelis, C. Xiong, M. Rother, T. Usbeck, Y. Yamazaki, J. Rauberg, and K. Styp-Rekowski (2021). Observing Earth’s magnetic environment with the GRACE-FO mission. Earth, Planets and Space, 73, 51. https://doi.org/10.1186/s40623-021-01364-w
-
I. Michaelis, K. Styp-Rekowski, J. Rauberg, C. Stolle, and M. Korte (2022). Geomagnetic data from the GOCE satellite mission. Earth, Planets, and Space, 74, 135. https://doi.org/10.1186/s40623-022-01691-6
-
K. Styp-Rekowski, I. Michaelis, C. Stolle, J. Baerenzung, M. Korte, and O. Kao (2022). Machine Learning-based Calibration of the GOCE Satellite Platform Magnetometers. Earth, Planets, and Space, 74, 138. https://doi.org/10.1186/s40623-022-01695-2
Conference presentations
- K. Styp-Rekowski, C. Stolle, O. Kao, and I. Michaelis. Satellite Platform Magnetometer Calibration Using Machine Learning. (Oral Presentation), Joint Scientific Assembly IAGA-IASPEI, Online, 21-27 August 2021.
- K. Styp-Rekowski, C. Stolle, O. Kao, and I. Michaelis. Automatic Calibration of Satellite Platform Magnetometers with Neural Network-based Time Shift Approximation. (Oral Presentation), Joint Scientific Assembly IAGA-IASPEI, Online, 21-27 August 2021.
- K. Styp-Rekowski, C. Stolle, I. Michaelis, and O. Kao. Machine Learning-based Information Extraction from Non-dedicated Sensors. (Oral Presentation), Photonics Days Berlin Brandenburg, Berlin, Germany, 4-7 October 2021.
- K. Styp-Rekowski, C. Stolle, I. Michaelis, and O. Kao. Calibration of GRACE-FO and GOCE Platform Magnetometers Using Machine Learning. (Oral Presentation), Swarm Data Quality Workshop, Athens, Greece, 11-16 October 2021.
- K. Styp-Rekowski, C. Stolle, I. Michaelis, and O. Kao. Calibration of the GRACE-FO Satellite Platform Magnetometers and Co-Estimation of Intrinsic Time Shift in Data. (Oral Presentation), IEEE Big Data 2021, Online, 15-18 December 2021.
- K. Styp-Rekowski, I. Michaelis, C. Stolle, and O. Kao. Magnetic Datasets from Non-dedicated Satellites. (Oral Presentation), Living Planet Symposium, Bonn, Germany, 23-27 May 2022.
- K. Styp-Rekowski, I. Michaelis, C. Stolle, and O. Kao. Physics-informed Neural Network for Platform Magnetometer Calibration. (Oral Presentation), Swarm Data Quality Workshop, Uppsala, Sweden, 10-14 October 2022.
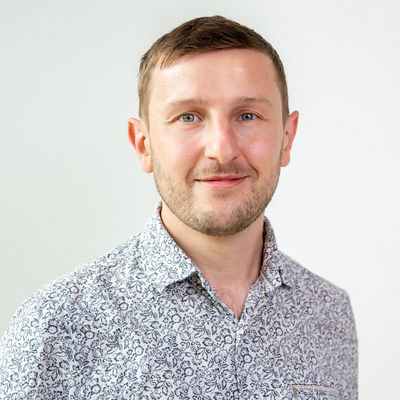
Mario Sänger
HU Berlin
Contact
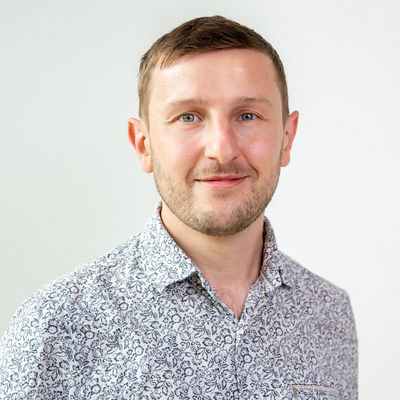
Supervisors:
Researchers are currently producing so many publications that it is impossible to keep up with the boom of discoveries even within a single field. Biomedical information extraction (IE) encompasses methods that aim to automatically collect biomedical knowledge from the scientific literature. These techniques are considered crucial for efficient access to published results at a scale that can cope with scientific progress. IE plays is essential in database curation, the construction of comprehensive models of pathways and cells, and fields such as Personalised Medicine. A key task for IE is the extraction of relationships between entities, such as drugs or proteins that interact with each other in a pathway or cell. While considerable progress in IE has been made over the two decades, there are deficits. Almost all the techniques have focused on extracting relationships from single sentences or single articles.
All sentence- and article-based methods suffer from a number of severe disadvantages in terms of design. First, a single record rarely provides enough evidence to establish the biological validity of a relationship, as the experimental evidence might be weak, or limited to a very specific context. Statements in texts may be more speculative than confirmative, and different articles often contradict each other. Experts therefore usually (a) try to acquire a comprehensive picture of the published state-of-the-art for any given question, and (b) need to include information from other sources in making informed decisions about relationships. There is no consensus on the best way to achieve this automatically. A solution will require finding suitable ways to encode the knowledge contained in large collections of texts and design efficient approaches to integrate different kinds of information (e.g. textual, numerical, categorical and molecular data) that originates from various sources.
This PhD project will contribute to this question while examining, harnessing and combining multiple information sources, such as the entire corpus of literature available through PubMed and additional knowledge base information, in hopes of improving the extraction of information on biomedical relationships. Our approach is fundamentally different than traditional approaches. We classify relations on a global, corpus-based level instead of the sentence- or article-based approaches currently in use. In particular, we want to explore representation learning techniques: instead of explicitly, manually modelling the connections between biomedical concepts, we will apply methods capable of learning adequate representations for these concepts by exploring correlations in large collections of (textual) data.
Full-length publications
- M. Sänger, and U. Leser (2020). Large-scale entity representation learning for biomedical relationship extraction. Bioinformatics, btaa674. https://doi.org/10.1093/bioinformatics/btaa674
- M. Kittner, M. Lamping, D. Rieke, J. Götze, B. Bajwa, I. Jelas, G. Rüter, H. Hautow, M. Sänger, ..., and U. Leser (2021). Annotation and initial evaluation of a large annotated German oncological corpus. JAMIA Open, 4(2), ooab025. https://doi.org/10.1093/jamiaopen/ooab025
- L. Weber, M. Sänger, J. Münchmeyer, M. Habibi, U. Leser, and A. Akbik (2021). HunFlair: An easy-to-use tool for state-of-the-art biomedical named entity recognition. Bioinformatics, btab042. https://doi.org/10.1093/bioinformatics/btab042
- L. Weber, M. Sänger, S. Garda, F. Barth, C. Alt, and U. Leser (2021). Humboldt @ DrugProt: Chemical-protein relation extraction with pretrained transformers and entity descriptions. In Proceedings of the 7th BioCreative Challenge Evaluation Workshop.
- L. Weber, M. Sänger, S. Garda, F. Barth, C. Alt, and U. Leser (2022). Chemical-Protein Relation Extraction with Ensembles of Carefully Tuned Pretrained Language Models. Database. https://doi.org/10.1093/database/baac098
-
J. Fries, L. Weber, N. Seelam, G. Altay, D. Datta, S. Garda, .. , M.Sänger, … , B. Beilharz (2022). Bigbio: a framework for data-centric biomedical natural language processing. Advances in Neural Information Processing Systems, 35, 25792-25806.
-
M. Sänger, N. De Mecquenem, K.E. Lewińska, V. Bountris, F. Lehmann, U. Leser, T. Kosch (2023). Large Language Models to the Rescue: Reducing the Complexity in Scientific Workflow Development Using ChatGPT. arXiv arXiv:2311.01825 [Preprint]
Conference presentations
- J. Seva, M. Sänger and U. Leser. Language-independent ICD-10 Coding using Multi-lingual Embeddings and Recurrent Neural Networks. (Oral presentation), CLEF eHealth 2018.
- M. Sänger, L. Weber, M. Kittner and U Leser. Classifying German Animal Experiment Summaries with Multi-lingual BERT. (Oral presentation), CLEF eHealth 2019.
- M. Saenger, L. Weber and U. Leser. WBI at MEDIQA 2021: Summarizing Consumer Health Questions with Generative Transformers. BioNLP Workshop - MEDIQA, 11 June 2021. https://www.aclweb.org/anthology/2021.bionlp-1.9.pdf
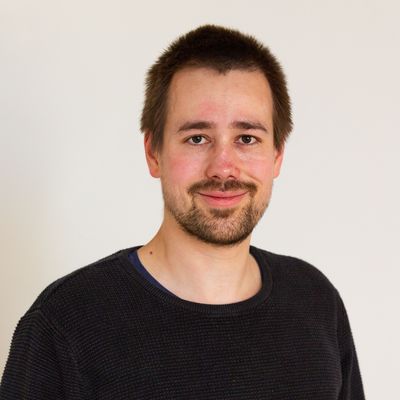
Peter Tillmann
HZB - FU Berlin
Contact
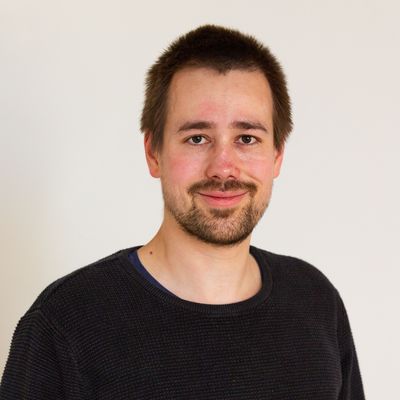
Supervisors:
Currently, perovskite-silicon (pero-Si) tandem solar cells are the most investigated concept to overcome the theoretical limit for the power conversion efficiency of single-junction silicon solar cells, with is 29.4%. Optical simulations are extremely valuable to study the distribution of light within the solar cells, and allow to minimize losses from reflection and parasitic absorption. For monolithic perovskite-silicon solar cells, it is vital that the available light is equally distributed between the two subcells, which is known as current matching. Nanotextures have proven to strongly reduce reflective losses. In this project we investigate, how realistic weather conditions affect the performance of pero-Si modules. We study, how different light management approaches, such as pyramidal texturing or (sinusoidal) nanotexturing influence the sensitivity of the solar module to the illumination condition. In contrast to single-junction silicon solar cells, (two-terminal) tandem solar cells are more sensitive to the spectral distribution of the incident light.
Doctoral Thesis
P. Tillmann (2023). Optimizing Bifacial Tandem Solar Cells for Realistic Operation Conditions Freie Universität Berlin. http://dx.doi.org/10.17169/refubium-39571
Full-length publications
- P. Tillmann, K. Jäger, and C. Becker (2020). Minimising the levelised cost of electricity for bifacial solar panel arrays using Bayesian optimization. Sustainable Energy Fuels, 4, 254-264. https://doi.org/10.1039/C9SE00750D
- K. Jäger, P. Tillmann, E.A. Katz, and C. Becker (2020). Perovskite/silicon tandem solar cells: Effect of luminescent coupling and bifaciality. Sol. RRL. https://doi.org/10.1002/solr.202000628
- K. Jäger, P. Tillmann, and C. Becker (2020). Detailed illumination model for bifacial solar cells. Opt. Express, 28, 4, 4751-4762. https://doi.org/10.1364/OE.383570
- P. Tillmann, B. Bläsi, S. Burger, M. Hammerschmidt, O. Höhn, C. Becker, and K. Jäger (2021). Optimizing metal grating back reflectors for III-V-on-silicon multijunction solar cells. Opt. Express, 29, 22517. https//doi.org/10.1364/OE.426761
- P. Tillmann, K. Jäger, A. Karsenti, L. Kreinin, and C. Becker (2022). Model-Chain Validation for Estimating the Energy Yield of Bifacial Perovskite/Silicon Tandem Solar Cells. Sol. RRL, 202200079. https://doi.org/10.1002/solr.202200079
Conference presentations
- P. Tillmann, C. Becker, and K. Jäger. Analysing the angular reflection losses of bifacial solar cells. (Poster presentation), European Photovoltaic Solar Energy Conference and Exhibition (EU PVSEC), Online, 7-11 September 2020.
- P. Tillmann, K. Jäger, E.A. Katz, and C. Becker. Relaxed current-matching constraints in perovskite/silicon tandem solar cell by bifacial operation and luminescent coupling. (Oral presentation), IEEE Photovoltaic Specialists Conference (PVSC), Online, 20-25 June 2021.
- P. Tillmann, K. Jäger, A. Karsenti, L. Kreinin, and C. Becker. Validation of Energy Yield Model for Bifacial Solar Cells and Prediction of Perovskite/silicon Tandem Solar Cell Performance. (Poster presentation), TandemPV, Freiburg, Germany, 30 May - 1 June 2022.
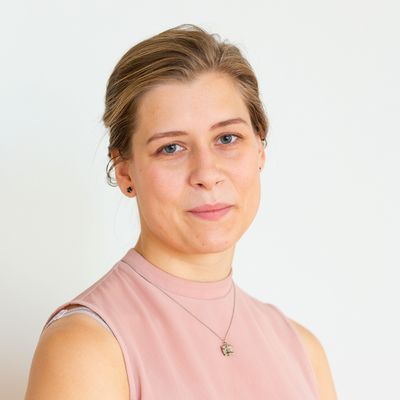
Anna Vlot
MDC - Uni Tübingen
Contact
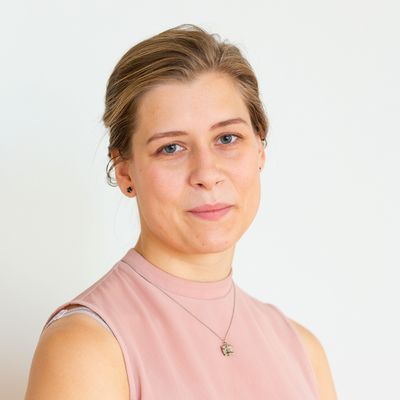
Supervisors:
Setareh Maghsudi (Uni Tübingen)
Cells are the building blocks of all multicellular organisms. Generally speaking, the DNA in each cell in a single organism is identical. Yet each different type of cell has its specialized function. These functional differences occur because cells of a particular identity transcribe a distinct set of genes into RNA molecules, many of which the cell then translates into proteins that determine cell structure, function, and identity. We do not yet fully understand the mechanisms that determine which genes and proteins a given cell produces. What we do know, however, is that the packing of DNA into a structure called chromatin plays a role. It is this packing that permits a 2-meter-long strand of DNA to fit into a cell nucleus with a diameter of no more than roughly 6 micrometres. If a gene lies in a region of the DNA that is tightly packed, the gene is not accessible for binding by the molecules that govern its transcription into RNA molecules. Thus, genes in inaccessible chromatin regions are not transcribed into RNA. However, protein-encoding regions make up just 2% of the human genome, and the accessibility of genomic regions alone does not explain cell-to-cell differences. Namely, non-protein-coding regions of the DNA, e.g. cis-regulatory regions, regulate gene expression. These regions, too, cannot exert their function if they are not accessible. Ultimately, the abundance of particular RNAs and the accessibility of chromatin together provide a starting point for unravelling the processes underlying cell identity acquisition and cell function.
Recently, researchers have begun measuring RNA abundance, chormatin accessibility, and more, in individual cells using so called single-cell omics assys. Analysis of the data obtained from these single-cell omics assays may provide novel insights into how cells aquire their identity. However, analysis of this data is complicated by its high-dimensional, sparse, and noisy nature. High dimensionality refers to the fact that tens of thousands of genes or hundreds of thousands of DNA region are measured in thousands to millions of cells. Sparsity occurs because most genes are not expressed in any given cell, and most regions of chromatin are not accessible. Besides, due to technical limitations, not all genes that are expressed or chromatin regions that are accessible in a given cell are captured. The combination of inherent sparsity and futher technical limitations results in noisy data with a poor signal-to-noise ratio. Taken together, these data characteristics complicate the identifcation of biologically meaningful patterns from the data, especially for genes that expressed at very low levels, or in only a few cells. This is of particular concern when considering cells at different stages of development since differences between cells may be restricted to the expression of only a few genes or subtle changes in chromatin accessibility.
In this project, we aim to develop methods to identify RNA molecules and cis-regulatory regions that characterize cell types and regulate the acquisition of cell identity. For this, we will adapt existing analytical approaches for the analysis of data representing continuous differentiation processes, without discretizing cells indetities into distinct cell states. This criterion is essential if we hope to identify genes and cis-regulatory regions that govern the development of cells in health and disease, where disease occurs due to abberent cell functions induced by disregulation of gene expression.
Doctoral thesis
Vlot AHC. (2023). Identifying markers of cell identity from single cell omics data. Humboldt Universität Berlin. doi:10.18452/27236
Full-length publications
- P. Rautenstrauch, A.H.C. Vlot, S. Saran, and U. Ohler (2021). Intricacies of single-cell multi-omics data integration. Trends in Genetics. https://doi.org/10.1016/j.tig.2021.08.012
- R. Shahan, C.W. Hsu, T.M. Nolan, B.J. Cole, I.W. Taylor, A.H.C. Vlot, P.N. Benfey, and U. Ohler (2022). A single cell Arabidopsis root atlas reveals developmental trajectories in wild type and cell identity mutants. Developmental Cell 57(4), 543-560.e9. https://doi.org/10.1016/j.devcel.2022.01.008
- A.H.C. Vlot, S. Maghsudi, and U. Ohler (2022). Cluster-independent marker feature identification from single-cell omics data using SEMITONES. Nucleic Acids Research, gkac639. https://doi.org/10.1093/nar/gkac639
Conference presentations
- R. Shahan, C.W. Hsu, T.M. Nolan, B.J. Cole, I.W. Taylor, A.H.C. Vlot, P.N. Benfey, and U. Ohler (2020). A single cell Arabidopsis root atlas reveals developmental trajectories in wild type and cell identity mutants. bioRxiv 2020.06.29.178863. https://doi.org/10.1101/2020.06.29.178863
-
A.H.C. Vlot, S. Maghsudi, and U. Ohler. Identification of marker genes and cis-regulatory regions using Single-cEll Marker IdentificaTiON by Enrichment Scoring (SEMITONES). (Poster presentation), 13th annual RECOMB/ISCB Conference on Regulatory & Systems Genomics with DREAM Challenges, Online, 16-19 November 2020.
-
A.H.C. Vlot, S. Maghsudi, and U. Ohler. Single-cEll Marker IdentificaTiON by Enrichment Scoring. (Poster and oral presentation), ISMB/ECCB 2021, Online, 25-30 July 2021.
- A.H.C. Vlot, S. Maghsudi, and U. Ohler. Identification of cis-regulatory regions using Single-cEll Marker IdentificaTiON by Enrichment Scoring (SEMITONES). (Poster presentation), EMBO Workshop Enhanceropathies: Understanding enhancer function to understand human disease, 6-9 October 2021.
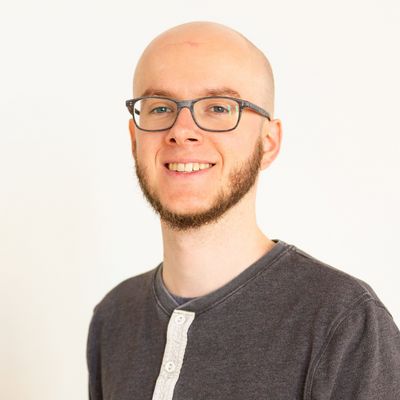
Leon Weber
HU Berlin - MDC
Contact
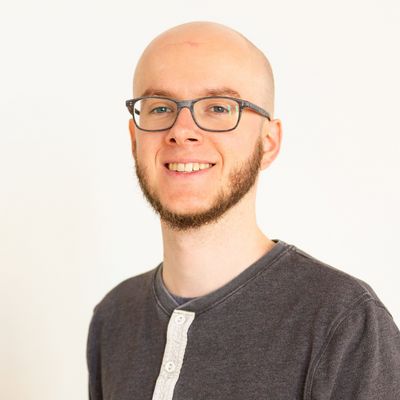
Supervisors:
Current attempts to decipher the molecular basis of cellular processes and human diseases are based on quantitative or qualitative models of the complex interplay between molecules in the cell, for instance in gene regulation, cellular signaling, or the metabolism. Obtaining such models in sufficient quality and breadth is a laborious task which today is predominantly based on human experts manually searching and reading the scientific literature with the aim to collect the many dispersed pieces of knowledge necessary to derive at a comprehensive picture. This work can be supported by using Text Mining, however, current research in this area focuses on extracting information from isolated sentences, which often produces unsatisfactory results as important contextual information is ignored (such as the experimental evidence of a reported fact, the precise species in which a finding was experimentally observed, the strength of the observed effects, possible previous treatments (with certain drugs) of the experimental system etc.). In this PhD project, we follow a radically different approach. We use the entire corpus of available scientific publications (roughly 30 Million abstracts, 1.5 Million full texts, possibly patents) as the source of inference for single relationships. To this end, a machine learning setup will be designed, where models of valid relationships are learned from all mentions of their constituents trained on a set of proven relationships. We use that approach to significantly expand the molecular network of several clinically relevant molecular pathways of which the PIs have comprehensive background knowledge, such as NF-kB signaling pathway, a pathway that is critically involved in cell faith decisions and perturbed in a number of diseases including cancer and inflammatory diseases, and the p53 pathway, which is strongly perturbed in cancer. The central aim of the PhD project is the extension of the currently available restricted pathway models, however, additional directions of expansion will also be investigated, such as development of cell-type -specific models, or elucidation of cross-talk to other pathways. We also envision using the new method to study connections between signaling pathways and existing targeted cancer therapies, for which patent texts would be extremely useful. Results from such text mining algorithms will be rigorously assessed in terms of their quality and relevance for biomedical research by (a) qualitatively checking the results at the literature level, and (b) quantitatively evaluating the performance of the expanded or improved pathways in typical analysis settings using OMICS data, such as pathways enrichment analysis and predictive power for selected phenotypes. The approach would allow a new way of predicting treatments that ideally can be adapted and specified for subgroups harboring individual combinations of perturbations in the disease-relevant pathways.
Doctoral thesis
L. Weber (2023). Text Mining for Pathway Curation. Humboldt Universität Berlin. doi: 10.18452/27520
Full-length publications
- L. Weber, J. Münchmeyer, T. Rocktäschel, M. Habibi, and U. Leser (2019). HUNER: Improving biomedical NER with pretraining. Bioinformatics, 36(1), 295-302. 10.1093/bioinformatics/btz528
- L. Weber, P. Minervini, J. Münchmeyer, U. Leser, and T. Rocktäschel (2019). NLProlog: Reasoning with weak unification for question answering in Natural Language. In Proceedings of the 57th Annual Meeting of the Association for Computational Linguistics, 6151-6161. 10.18653/v1/P19-1618
- L. Weber, K. Thobe, O.A.M. Lozano, J. Wolf, and U. Leser (2020). PEDL: Extracting protein-protein associations using deep language models and distant supervision. Bioinformatics, 36(1), i490–i498. https://doi.org/10.1093/bioinformatics/btaa430
- W.D. Xing, L. Weber, and U. Leser (2020). Biomedical event extraction as multi-turn question answering. In Proceedings of the 11th Int. Workshop on Health Text Mining and Information Analysis, 88-96. 10.18653/v1/2020.louhi-1.10
- L. Weber, M. Sänger, J. Münchmeyer, M. Habibi, U. Leser, and A. Akbik (2021). HunFlair: An easy-to-use tool for state-of-the-art biomedical named entity recognition. Bioinformatics, btab042. https://doi.org/10.1093/bioinformatics/btab042
- L. Weber, M. Sänger, S. Garda, F. Barth, C. Alt, and U. Leser (2021). Humboldt @ DrugProt: Chemical-protein relation extraction with pretrained transformers and entity descriptions. In Proceedings of the 7th BioCreative Challenge Evaluation Workshop.
- L. Weber, S. Garda, J. Münchmeyer, and U. Leser (2021). Extend, don’t rebuild: Phrasing conditional graph modification as autoregressive sequence labelling. In Proceedings of the 2021 Conference on Empirical Methods in Natural Language Processing, 1213–1224.
- K. Singh, J. Münchmeyer, L. Weber, U. Leser, and A. Bande (2022). Graph Neural Networks for Learning Molecular Excitation Spectra.J. Chem. Theory Comp., 18, 7, 4408-4417. DOI: 10.1021/acs.jctc.2c00255
- J.A. Fries, N. Seelam, G. Altay, L. Weber, M. Kang, D. Datta, R. Su, S. Garda, B. Wang, S. Ott, M. Samwald, and W. Kusa (2022). Dataset Debt in Biomedical Language Modeling. In Proceedings of the Workshop on Challenges & Perspectives in Creating Large Language Models, 137-145. https://doi.org/10.18653/v1/2022.bigscience-1.10
- X. Wang, U. Leser, and L. Weber (2022). BEEDS: Large-Scale Biomedical Event Extraction using Distant Supervision and Question Answering. In Proceedings of BioNLP, 298-309. 10.18653/v1/2022.bionlp-1.28
- L. Weber, M. Sänger, S. Garda, F. Barth, C. Alt and U. Leser (2022). Chemical-Protein Relation Extraction with Ensembles of Carefully Tuned Pretrained Language Models. Database, 2022, baac098. https://doi.org/10.1093/database/baac098
- J. Fries, L. Weber, N. Seelam, G. Altay, D. Datta, S. Garda, .. , M.Sänger, … , B. Beilharz (2022). Bigbio: a framework for data-centric biomedical natural language processing. Advances in Neural Information Processing Systems, 35, 25792-25806.
- H. Laurençon, L. Saulnier, T. Wang, C. Akik, A. V. del Moral, T. Le Scao, ... L. Weber, ... et al. (2022). The BigScience Corpus A 1.6 TB Composite Multilingual Dataset. https://openreview.net/forum?id=UoEw6KigkUn [Preprint]
- L. Weber, F. Barth, L. Lorenz, F. Konrath, K. Huska, J. Wolf, and U. Leser (2023). PEDL+: Protein-centered relation extraction from PubMed at your fingertip. Bioinformatics, 39, 11. doi:10.1093/bioinformatics/btad603
Conference presentations
- L. Weber, P. Minervini, J. Münchmeyer, U. Leser, and T. Rocktäschel. NLProlog: Reasoning with weak unification for question answering in Natural Language. (Poster presentation) 57th Annual Meeting of the Association for Computational Linguistics, Florence, Italy, 28 July - 2 August, 2019.
- M. Saenger, L. Weber, and U. Leser. WBI at MEDIQA 2021: Summarizing Consumer Health Questions with Generative Transformers. BioNLP Workshop - MEDIQA, 11 June 2021. https://www.aclweb.org/anthology/2021.bionlp-1.9.pdf